A black-box adversarial example generation method based on low query image data
A technology of adversarial samples and image data, applied in the field of adversarial sample generation, can solve problems such as low success rate and high number of iterations of adversarial samples, and achieve the effect of improving randomness, increasing the possibility of adversarial resistance, and improving evaluation efficiency
- Summary
- Abstract
- Description
- Claims
- Application Information
AI Technical Summary
Problems solved by technology
Method used
Image
Examples
Embodiment Construction
[0037] The present invention will be further described below in conjunction with the accompanying drawings and specific embodiments.
[0038] In related applications of image recognition, the present invention combines the advantages of migration-based and optimization-based generation methods, and reduces the number of iterations, that is, the number of inquiries while ensuring the success rate of generating black-box confrontation samples. The main improvement method of the present invention is to modify the random vector added in each iteration in the traditional method into an adversarial vector, and at the same time, increase the randomness in the process of generating the adversarial vector to avoid falling into local optimum and further improve production efficiency.
[0039]The image data set used in this experiment is from http: / / www.image-net.org / , and the data set uses ImageNet. The data of this experiment is randomly obtained from this data set. Each time the input...
PUM
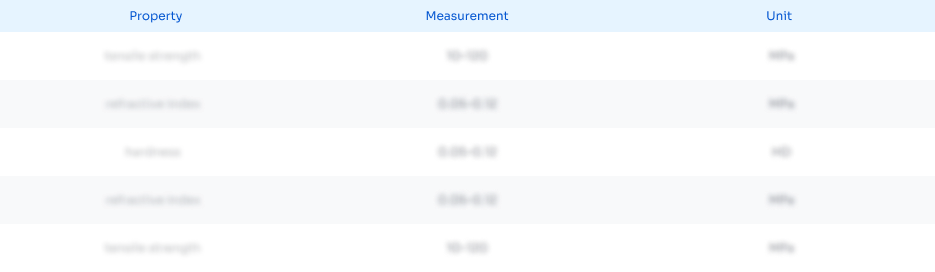
Abstract
Description
Claims
Application Information
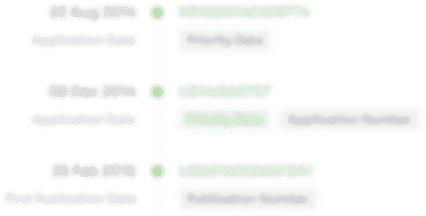
- R&D Engineer
- R&D Manager
- IP Professional
- Industry Leading Data Capabilities
- Powerful AI technology
- Patent DNA Extraction
Browse by: Latest US Patents, China's latest patents, Technical Efficacy Thesaurus, Application Domain, Technology Topic.
© 2024 PatSnap. All rights reserved.Legal|Privacy policy|Modern Slavery Act Transparency Statement|Sitemap