Method for constructing health assessment iterative classifier model
A technology of health assessment and construction method, which is applied in the direction of health index calculation, neural learning method, biological neural network model, etc., can solve problems such as dependence on the degree of understanding, achieve good generalization ability, improve physical and mental health, and speed up the effect
- Summary
- Abstract
- Description
- Claims
- Application Information
AI Technical Summary
Problems solved by technology
Method used
Image
Examples
Embodiment 1
[0041] A method for constructing a health assessment iterative classifier model, such as figure 1 , figure 2 As shown, collect health data and determine the numerical interval standard of each influencing factor, then divide the collected health data according to the numerical interval standard, and mark the categories; use the fuzzy set membership function to determine the degree of influence of each factor on the user's health, A training sample set required for training is formed; an iterative classifier model is trained, and the iterative classifier model adopts several weak classifiers to integrate a strong classifier, and the weak classifier adopts a BP neural network structure.
[0042] The iterative classifier adopts the integration of multiple weak classifiers to form a strong classifier, which is not easy to cause over-fitting in the training process. The BP neural network can have a certain fault-tolerant ability to unprocessed noise, and has good generalization a...
Embodiment 2
[0044] This embodiment is optimized on the basis of embodiment 1, such as figure 2 As shown, the fuzzy set membership function calculates the probability distribution of each data point through the Gaussian mixture model, and then assigns different membership degrees to different data points according to the obtained probability distribution. The invention proposes to establish a corresponding degree of membership for each factor affecting health, which can determine the degree of influence of each factor on health and speed up the speed of discovering existing problems.
[0045] At present, most researches on fuzzy membership function are based on the distance measurement membership degree constructed based on the distance from the sample point to the category center point. The closer the distance to the center point, the higher the membership degree, and its design directly affects the performance of the classifier. If there are discrete points in the data, the membership fun...
Embodiment 3
[0048] This embodiment is optimized on the basis of embodiment 1 or 2, as image 3 As shown, training an iterative classifier model mainly includes the following steps:
[0049] Step S100: Load the training sample set, initialize the weight of the classifier model, and train the weak classifier 1;
[0050] Step S200: Train the weak classifier according to the set number of iterations, and calculate the error rate e when the maximum number of iterations is reached t , update weight α t ;
[0051] Step S300: use the updated weight parameters to train the weak classifier 2;
[0052] Step S400: Repeat steps S100-S300 until all weak classifiers are trained;
[0053] Step S500: Using a combination strategy to integrate all weak classifiers into a strong classifier, and perform an accuracy test on the strong classifier, and select an optimal classification model.
[0054] Further, first calculate the average precision of each weak classification model after t iterations, and use...
PUM
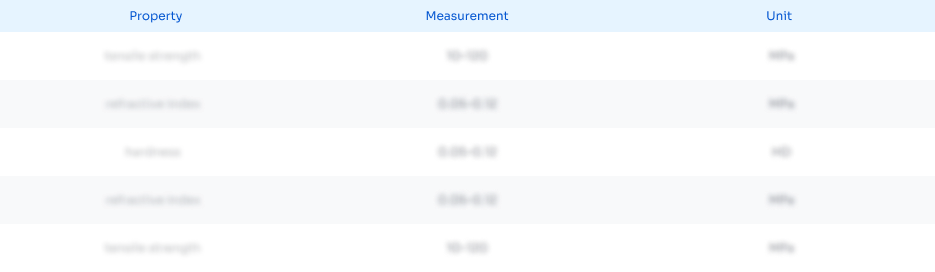
Abstract
Description
Claims
Application Information
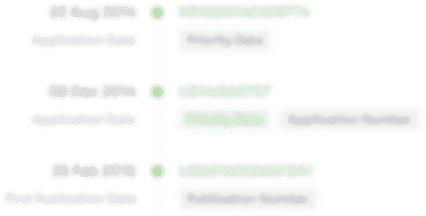
- R&D Engineer
- R&D Manager
- IP Professional
- Industry Leading Data Capabilities
- Powerful AI technology
- Patent DNA Extraction
Browse by: Latest US Patents, China's latest patents, Technical Efficacy Thesaurus, Application Domain, Technology Topic, Popular Technical Reports.
© 2024 PatSnap. All rights reserved.Legal|Privacy policy|Modern Slavery Act Transparency Statement|Sitemap|About US| Contact US: help@patsnap.com