Mechanical assembly image segmentation method and equipment based on deep learning network
A deep learning network and image segmentation technology, which is applied in the fields of computer image recognition and intelligent manufacturing, can solve problems such as single color and texture information of mechanical parts, serious parts occlusion, and complex structure of mechanical products, so as to improve segmentation performance and reduce the number of parameters Effect
- Summary
- Abstract
- Description
- Claims
- Application Information
AI Technical Summary
Problems solved by technology
Method used
Image
Examples
Embodiment 1
[0034] see figure 1 , a method for image segmentation of mechanical assemblies based on a deep learning network, comprising the following steps:
[0035] A lightweight semantic segmentation model of a mechanical assembly composed of an encoder network and a decoder network is constructed through a deep learning network; in this embodiment, the deep learning neural network is based on the Unet model.
[0036] To establish a mechanical assembly data set, first establish a plurality of three-dimensional models of mechanical assemblies through SolidWorks, and add color marks to each part in each of the three-dimensional models. Batch 3D rendering of the above 3D models to obtain the depth images and corresponding label images of the 3D models at different angles; select a depth image in the assembly stage from each 3D model, integrate it into a test set, and use the remaining depth images as a training set; The embodiment data set is composed of depth images of different assembly...
Embodiment 2
[0058] A mechanical assembly image segmentation device based on a deep learning network, including a memory, a processor, and a computer program stored in the memory and operable on the processor. described method.
Embodiment 3
[0060] A mechanical assembly image segmentation medium based on a deep learning network, on which a computer program is stored, and when the program is executed by a processor, the method as described in the first embodiment is implemented.
PUM
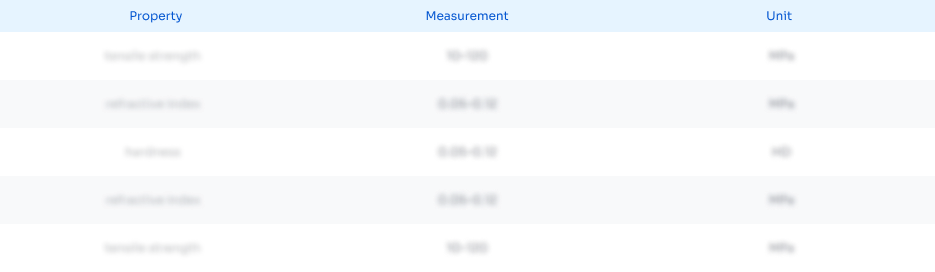
Abstract
Description
Claims
Application Information
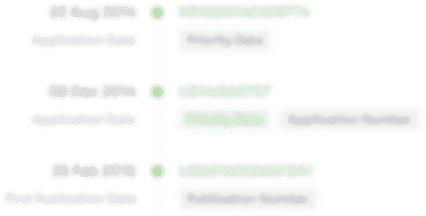
- R&D
- Intellectual Property
- Life Sciences
- Materials
- Tech Scout
- Unparalleled Data Quality
- Higher Quality Content
- 60% Fewer Hallucinations
Browse by: Latest US Patents, China's latest patents, Technical Efficacy Thesaurus, Application Domain, Technology Topic, Popular Technical Reports.
© 2025 PatSnap. All rights reserved.Legal|Privacy policy|Modern Slavery Act Transparency Statement|Sitemap|About US| Contact US: help@patsnap.com