Meta-learning-based domain increment method
A meta-learning and domain technology, applied in the domain incremental domain based on meta-learning, which can solve the problems of reduced model accuracy, difficulty in reconciliation, large data storage and training time overhead, etc., to achieve the effect of ensuring accuracy and reducing overhead.
- Summary
- Abstract
- Description
- Claims
- Application Information
AI Technical Summary
Problems solved by technology
Method used
Image
Examples
Embodiment
[0029] In this embodiment, a batch of old data D is first given old ,D old Composed of Mobike and Golden Retriever, and later a batch of new data D new ,D new Consisting of little yellow cars and huskies, the goal of this embodiment is to achieve a high accuracy rate for both old and new data. Such as figure 1 As shown, the method of this embodiment is as follows:
[0030] S1. Build a pre-training model: use the meta-learning method iTAML to select several public datasets as metadata, construct meta-tasks and learn a pre-training model, such as selecting airplanes and birds in the cifar10 dataset (task 1), driving a truck and Deer (task 2), car and horse (task 3), the classification model structure selects MobileNetV2, obtains the parameter φ of described pre-training model, and described pre-training model is convolution neural classification network, and it should be pointed out that iTAML is the same as Model-independent, you can choose any convolutional neural classif...
PUM
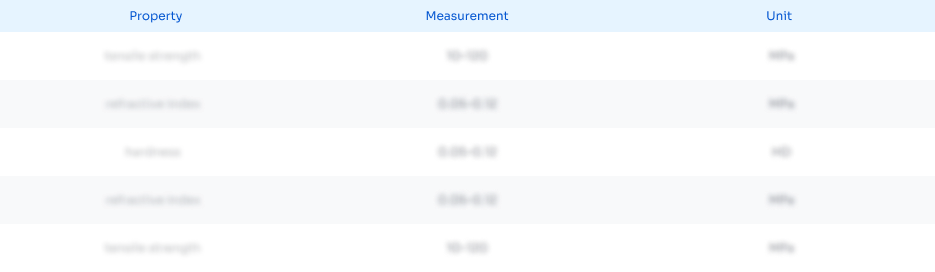
Abstract
Description
Claims
Application Information
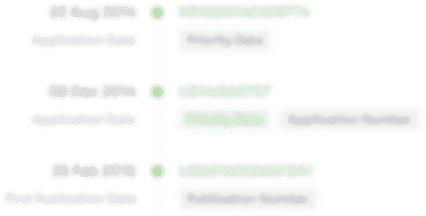
- R&D
- Intellectual Property
- Life Sciences
- Materials
- Tech Scout
- Unparalleled Data Quality
- Higher Quality Content
- 60% Fewer Hallucinations
Browse by: Latest US Patents, China's latest patents, Technical Efficacy Thesaurus, Application Domain, Technology Topic, Popular Technical Reports.
© 2025 PatSnap. All rights reserved.Legal|Privacy policy|Modern Slavery Act Transparency Statement|Sitemap|About US| Contact US: help@patsnap.com