Fine-grained entity classification method based on diversified semantic attention model
A technology of attention model and classification method, which is applied in the field of fine-grained entity classification based on diversified semantic attention model, and can solve the problem of low accuracy of fine-grained entity classification
- Summary
- Abstract
- Description
- Claims
- Application Information
AI Technical Summary
Problems solved by technology
Method used
Image
Examples
Embodiment
[0109] In order to verify the effectiveness of the solution of the present invention, the following simulation experiments are carried out.
[0110] Step 1: Generate attention segments. For example, the input sentence is: Skeptics wonder whether mega-resorts such as the Mirage will be able to squeeze a profit from their cashflow. According to the segmentation length and segmentation step size set in step 1, the input sentence can be segmented into: Skeptics wonder whether mega-resorts such as, wonder whether mega-resorts such as the Mirage will be able to squeeze a profit, the Mirage will be able to squeeze a profit from their cash flow and other sentences.
[0111] Step 2: Perform contextualized word vector encoding on the multiple sentences segmented in step 1. The example sentence in step 1 is used for illustration. The word vector encoding of the sentence is [-0.13128 -0.452 0.043399 -0.99798 -0.21053 -0.95868 -0.24609 0.48413 0.18178 0.475 -0.22305 0.30064 0.43496 -0.36...
PUM
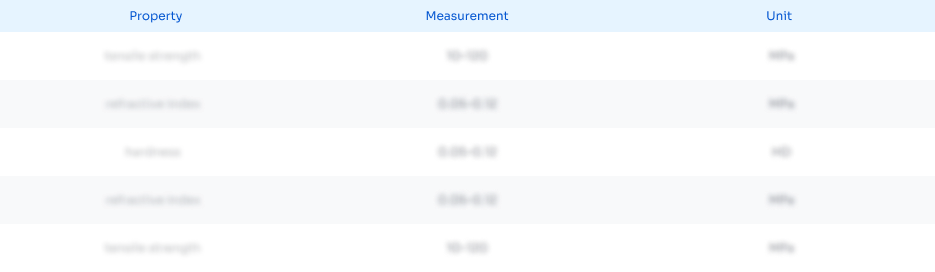
Abstract
Description
Claims
Application Information
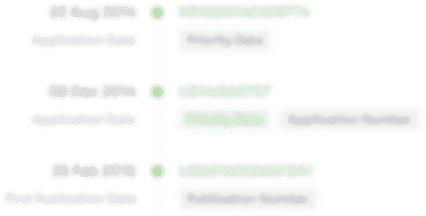
- R&D Engineer
- R&D Manager
- IP Professional
- Industry Leading Data Capabilities
- Powerful AI technology
- Patent DNA Extraction
Browse by: Latest US Patents, China's latest patents, Technical Efficacy Thesaurus, Application Domain, Technology Topic, Popular Technical Reports.
© 2024 PatSnap. All rights reserved.Legal|Privacy policy|Modern Slavery Act Transparency Statement|Sitemap|About US| Contact US: help@patsnap.com