Online car-hailing target order prediction method based on CNN-LSTM
A forecasting method and online car-hailing technology, applied in forecasting, network data retrieval, network data indexing, etc., can solve problems that hinder the profitability of online car-hailing travel companies, difficulty in predicting target order volume, long empty mileage, etc.
- Summary
- Abstract
- Description
- Claims
- Application Information
AI Technical Summary
Problems solved by technology
Method used
Image
Examples
Embodiment Construction
[0061] In order to better understand the present invention, the invention will be described in detail below in conjunction with specific examples.
[0062] Combine figure 1 As shown, in Wuhan as an example:
[0063] S1: Split the preset area into several sub-regions;
[0064] GEOHASH-based grid division and number, GEOHASH encoding is a rectangular area, the larger the number of geohash encoding bits, the smaller the representative, and divide the Wuhan City, and divide it. , From north to south for regional naming;
[0065] Geohash divides the entire Wuhan map so that we can divide the entire map area into 108 regions, identifying these 108 regions by numbers.
[0066] S2: Collect the original order data of 108 regions in Wuhan:
[0067] Provided by the travel company, the original order data in Wuhan, the original order data includes the user ID, the driver ID, the order number, the user's order time, the driver picks up the unit, the driver's latitude latitude, the user's payme...
PUM
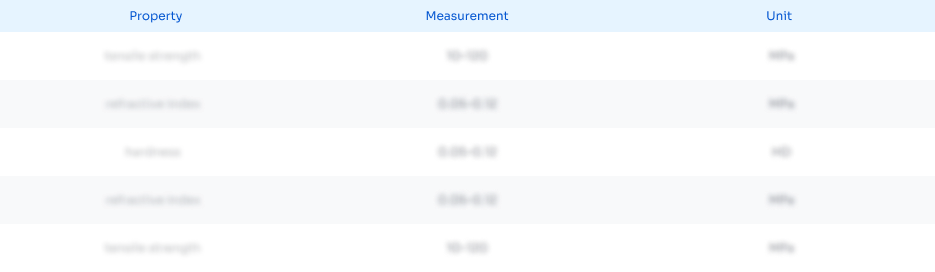
Abstract
Description
Claims
Application Information
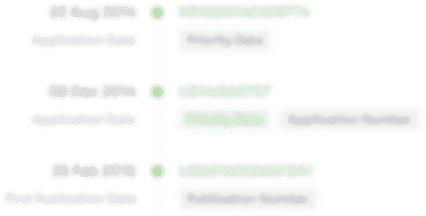
- R&D
- Intellectual Property
- Life Sciences
- Materials
- Tech Scout
- Unparalleled Data Quality
- Higher Quality Content
- 60% Fewer Hallucinations
Browse by: Latest US Patents, China's latest patents, Technical Efficacy Thesaurus, Application Domain, Technology Topic, Popular Technical Reports.
© 2025 PatSnap. All rights reserved.Legal|Privacy policy|Modern Slavery Act Transparency Statement|Sitemap|About US| Contact US: help@patsnap.com