Text multi-label classification method and system based on attention mechanism and GCN
A classification method and attention technology, applied in the direction of text database clustering/classification, neural learning method, unstructured text data retrieval, etc. Classification accuracy and other issues to achieve the effect of improving feature extraction capabilities
- Summary
- Abstract
- Description
- Claims
- Application Information
AI Technical Summary
Problems solved by technology
Method used
Image
Examples
Embodiment Construction
[0071] In order to make the object, technical solution and advantages of the present invention clearer, the present invention will be further described in detail below in conjunction with the accompanying drawings and embodiments. It should be understood that the specific embodiments described here are only used to explain the present invention, not to limit the present invention. In addition, the technical features involved in the various embodiments of the present invention described below can be combined with each other as long as they do not constitute a conflict with each other.
[0072] The basic idea of the present invention is to use the attention mechanism to construct the semantic correlation between texts, words, and tags. On the one hand, the text and tag information are summarized to form a new text word representation, and the text features are extracted more fully. On the other hand, On the one hand, text and word information are aggregated to form a new label...
PUM
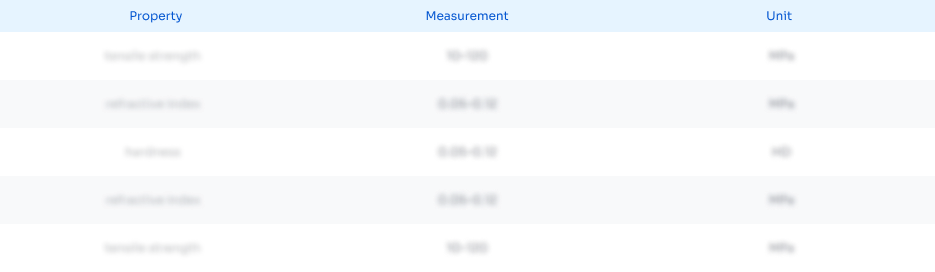
Abstract
Description
Claims
Application Information
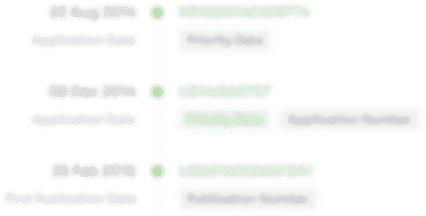
- R&D Engineer
- R&D Manager
- IP Professional
- Industry Leading Data Capabilities
- Powerful AI technology
- Patent DNA Extraction
Browse by: Latest US Patents, China's latest patents, Technical Efficacy Thesaurus, Application Domain, Technology Topic.
© 2024 PatSnap. All rights reserved.Legal|Privacy policy|Modern Slavery Act Transparency Statement|Sitemap