Hyperspectral image depth noise reduction method based on three-dimensional variational network
A hyperspectral image, three-dimensional variational technology, applied in the direction of probability network, image enhancement, image analysis, etc., can solve problems such as the inability to effectively promote and remove unknown noise, and achieve the effect of deep noise reduction
- Summary
- Abstract
- Description
- Claims
- Application Information
AI Technical Summary
Problems solved by technology
Method used
Image
Examples
Embodiment approach
[0053] The method for deep noise reduction of hyperspectral image based on three-dimensional variational network according to the present invention comprises the following steps: combining noise estimation and image denoising into a Bayesian inference framework, directly inferring the underlying noise from the noisy HSI The noise-free HSI image and the corresponding noise distribution, while considering the inherent spatial domain consistency and inter-spectral correlation of hyperspectral images, use three-dimensional convolution to extract spatial spectral features from multiple adjacent frequency bands. The specific process is as follows:
[0054] Model the noisy HSI linearly as:
[0055] Y=X+v (1)
[0056] where, Y, X, v∈R P×Q×N , Y, X, v represent noisy HIS, noise-free HIS and non-i.i.d. noise respectively, P, Q and N represent the space height, space width and the number of spectral bands respectively, by constructing the model shown in formula (2) To represent the gen...
Embodiment 1
[0105] 1. HSI denoising experiment based on Gaussian white noise
[0106] First, non-i.i.d. noise is added to the CAVE training set samples, where σ∈[0,75], to generate noisy HSI samples for training, and the noise of case1 is added to the ICVL test set for testing. Table 1 shows the denoising results under the noise level (σ=30,50,70) and Gaussian blind noise (σ∈[30,70]), while figure 2 The denoising result image of case1 noise with a variance of 50 added to the ICVL dataset is given. In order to better distinguish the details of the denoising result, the local area is enlarged as figure 2 shown. It can be clearly seen that the present invention is superior to other comparative methods in the three quantitative analysis methods and the denoising result graphs. Although methods like HSID-CNN and VDNet2D can suppress noise, the denoising results of the HSID-CNN method There are missing and blurred spatial textures in the image. For the VDNet2D method, some artifacts and res...
PUM
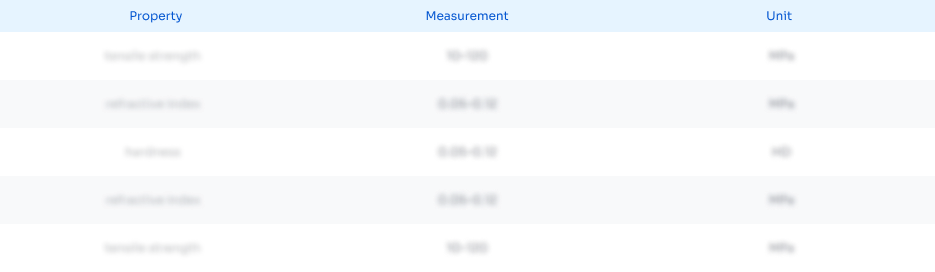
Abstract
Description
Claims
Application Information
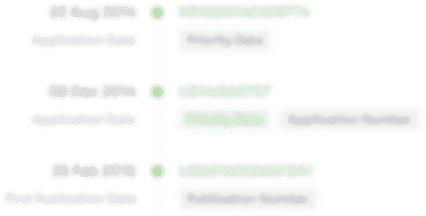
- R&D
- Intellectual Property
- Life Sciences
- Materials
- Tech Scout
- Unparalleled Data Quality
- Higher Quality Content
- 60% Fewer Hallucinations
Browse by: Latest US Patents, China's latest patents, Technical Efficacy Thesaurus, Application Domain, Technology Topic, Popular Technical Reports.
© 2025 PatSnap. All rights reserved.Legal|Privacy policy|Modern Slavery Act Transparency Statement|Sitemap|About US| Contact US: help@patsnap.com