Interpretable local migration mutual learning method based on attention map
A learning method and attention technology, applied in the field of image recognition, can solve problems such as poor model interpretability
- Summary
- Abstract
- Description
- Claims
- Application Information
AI Technical Summary
Problems solved by technology
Method used
Image
Examples
Embodiment Construction
[0045] The present invention will be further described below in conjunction with accompanying drawing.
[0046] Such as figure 1 As shown, the interpretable partial transfer and inter-learning method based on the attention map of the present invention mainly includes the steps of attention map construction, attention map similarity measurement, partial attention map transfer, and mutual transfer. The implementation method of the present invention will be described in detail from these aspects below.
[0047] Aiming at the problem that the feature map labeling area is inaccurate in the current model feature map visualization scheme, and labeling errors lead to a decrease in model interpretability. The present invention proposes an interpretable local transfer mutual learning algorithm based on an attention map. It mainly includes three parts: attention map construction algorithm, attention map similarity measurement algorithm and attention map local migration algorithm.
[0...
PUM
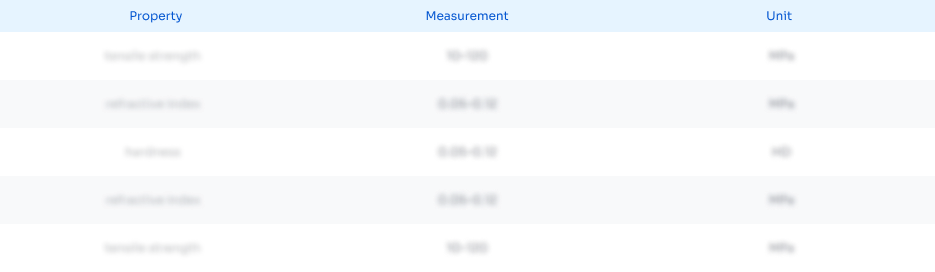
Abstract
Description
Claims
Application Information
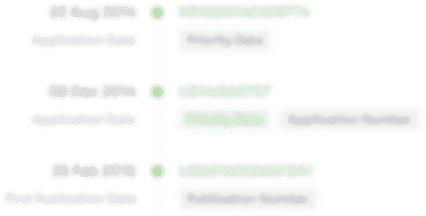
- R&D
- Intellectual Property
- Life Sciences
- Materials
- Tech Scout
- Unparalleled Data Quality
- Higher Quality Content
- 60% Fewer Hallucinations
Browse by: Latest US Patents, China's latest patents, Technical Efficacy Thesaurus, Application Domain, Technology Topic, Popular Technical Reports.
© 2025 PatSnap. All rights reserved.Legal|Privacy policy|Modern Slavery Act Transparency Statement|Sitemap|About US| Contact US: help@patsnap.com