Bus arrival time prediction method based on quantile convolutional network
A convolutional network, time prediction technology, applied in prediction, traffic flow detection, neural learning methods, etc., can solve the problems of shrinking passenger volume, random time distribution, lack of attractiveness, etc., to prevent gradient explosion and improve development. Effectiveness of utilization and improved accuracy
- Summary
- Abstract
- Description
- Claims
- Application Information
AI Technical Summary
Problems solved by technology
Method used
Image
Examples
Embodiment Construction
[0059] The present invention will be further described below in conjunction with specific embodiment:
[0060] Such as figure 1 As shown, a method for predicting bus arrival time based on the quantile convolutional network described in the embodiment of the present invention includes the following steps:
[0061] S1. Through the bus line information, road section information and bus GPS historical data collected in advance, the station number, the length between stations and the historical space-time location data of the bus operation are obtained after cleaning, and the cleaned data set is stored in mysql.
[0062] S2. Take 5 minutes as the time granularity to carry out data statistics on the travel time between bus stops, use y to represent the time required for the bus to drive back to the bus terminal in any GPS position, and use
[0063] x={x m ,x n ,x w ,x t ,x i ,x l ,x k ,x y} represents the covariate corresponding to y, where x m ∈[0,12] indicates that the t...
PUM
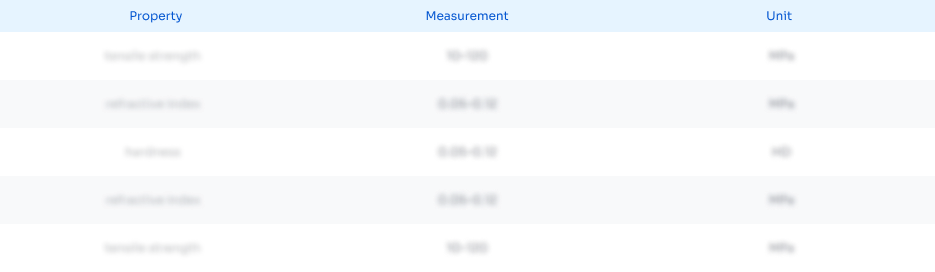
Abstract
Description
Claims
Application Information
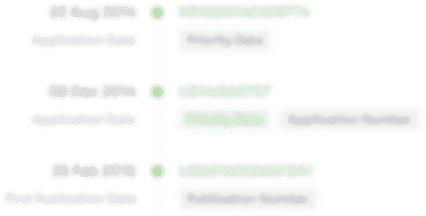
- R&D Engineer
- R&D Manager
- IP Professional
- Industry Leading Data Capabilities
- Powerful AI technology
- Patent DNA Extraction
Browse by: Latest US Patents, China's latest patents, Technical Efficacy Thesaurus, Application Domain, Technology Topic.
© 2024 PatSnap. All rights reserved.Legal|Privacy policy|Modern Slavery Act Transparency Statement|Sitemap