Meta-reinforcement learning-based vehicle adaptive autonomous driving decision-making method and system
An autonomous driving and reinforcement learning technology, applied in control/regulation systems, vehicle position/route/altitude control, non-electric variable control, etc., can solve problems such as unsafe decision-making, achieve flexibility, improve user experience, The effect of safe and stable driving process
- Summary
- Abstract
- Description
- Claims
- Application Information
AI Technical Summary
Problems solved by technology
Method used
Image
Examples
Embodiment Construction
[0053] Below in conjunction with specific embodiments, the present invention will be further illustrated, and it should be understood that these embodiments are only used to illustrate the present invention and not to limit the scope of the present invention. The modifications all fall within the scope defined by the appended claims of this application.
[0054]In the present invention, the selection of driving behavior is based on the task information given by the task coding module and the state information given by the perception module. The task to be completed is to quickly and safely reach another point on the map under a certain vehicle condition. Obviously, if a certain driving scheme can drive reliably under the current road conditions, the scheme will be given a positive reward value; otherwise, it will be given a negative reward value. To maximize cumulative reward, we need to find the optimal mapping from environmental states and task encodings to driving behavior....
PUM
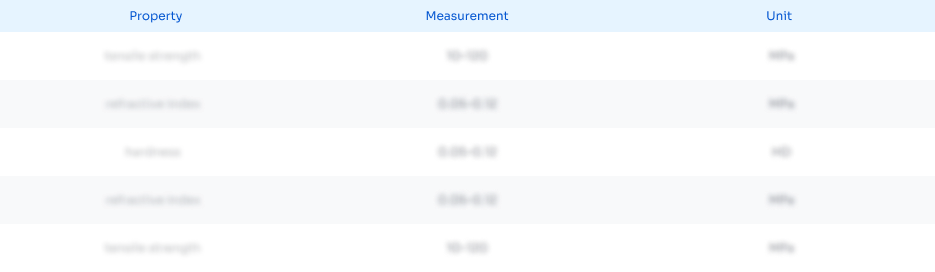
Abstract
Description
Claims
Application Information
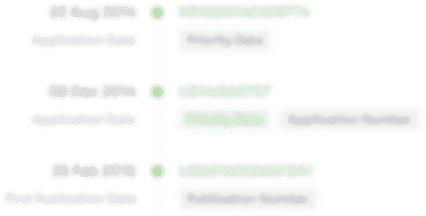
- R&D
- Intellectual Property
- Life Sciences
- Materials
- Tech Scout
- Unparalleled Data Quality
- Higher Quality Content
- 60% Fewer Hallucinations
Browse by: Latest US Patents, China's latest patents, Technical Efficacy Thesaurus, Application Domain, Technology Topic, Popular Technical Reports.
© 2025 PatSnap. All rights reserved.Legal|Privacy policy|Modern Slavery Act Transparency Statement|Sitemap|About US| Contact US: help@patsnap.com