Automatic driving decision-making method and system based on partial observable migration reinforcement learning
A reinforcement learning and automatic driving technology, applied in control/regulation systems, motor vehicles, non-electric variable control, etc., can solve problems such as unsatisfactory, and achieve the effect of improving utilization, wide application prospects, and strong robustness
- Summary
- Abstract
- Description
- Claims
- Application Information
AI Technical Summary
Problems solved by technology
Method used
Image
Examples
Embodiment Construction
[0067] Below in conjunction with specific embodiment, further illustrate the present invention, should be understood that these embodiments are only used to illustrate the present invention and are not intended to limit the scope of the present invention, after having read the present invention, those skilled in the art will understand various equivalent forms of the present invention All modifications fall within the scope defined by the appended claims of the present application.
[0068] In the present invention, the driving scheme is composed of observation set, driving strategy and termination function, and the task to be completed is to get from one point on the map to another point quickly and safely. Obviously, if a certain driving plan can be driven reliably under the current road conditions, the plan will be given a positive reward value; otherwise, a negative reward value will be given. To maximize the cumulative reward, we need to find the optimal mapping from road...
PUM
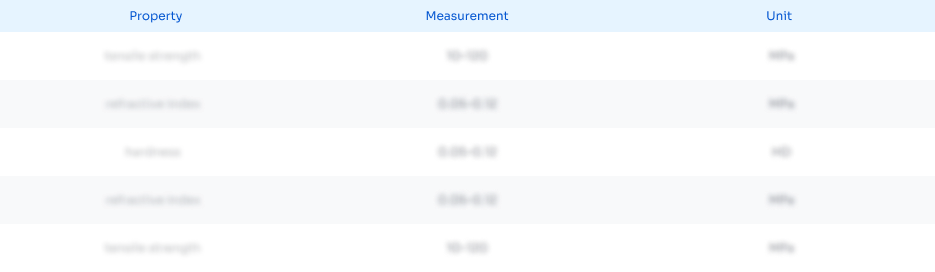
Abstract
Description
Claims
Application Information
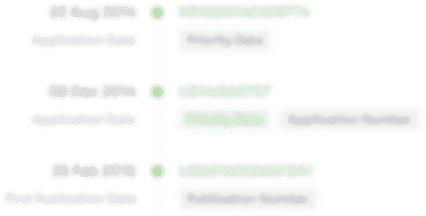
- R&D Engineer
- R&D Manager
- IP Professional
- Industry Leading Data Capabilities
- Powerful AI technology
- Patent DNA Extraction
Browse by: Latest US Patents, China's latest patents, Technical Efficacy Thesaurus, Application Domain, Technology Topic, Popular Technical Reports.
© 2024 PatSnap. All rights reserved.Legal|Privacy policy|Modern Slavery Act Transparency Statement|Sitemap|About US| Contact US: help@patsnap.com