Semantic segmentation with soft cross-entropy loss
A cross-entropy and semantic technology, applied in the field of machine learning and computer vision, can solve the problem that the mobile training environment is not very useful
- Summary
- Abstract
- Description
- Claims
- Application Information
AI Technical Summary
Problems solved by technology
Method used
Image
Examples
Embodiment Construction
[0017] Embodiments described below can be found in the disclosed systems and methods for semantic segmentation with soft cross-entropy loss. Exemplary aspects of the present disclosure provide a system that trains a semantic segmentation network suitable for real-time inference while maintaining a balance between classification accuracy and compactness of the semantic segmentation network. The disclosed system utilizes a soft cross-entropy (CE) loss as an auxiliary loss to regularize the training of semantic segmentation networks and reduce memory usage during training time. In contrast to conventional hard-label assignment for classification tasks, the disclosed system generates soft-assigned labels as a probability distribution at each auxiliary stride, and applies cross-entropy as an auxiliary loss function on soft targets. Here, soft assignments may differ from typical hard assignments, where each value of a feature map is assigned one of binary values (0 or 1). In soft...
PUM
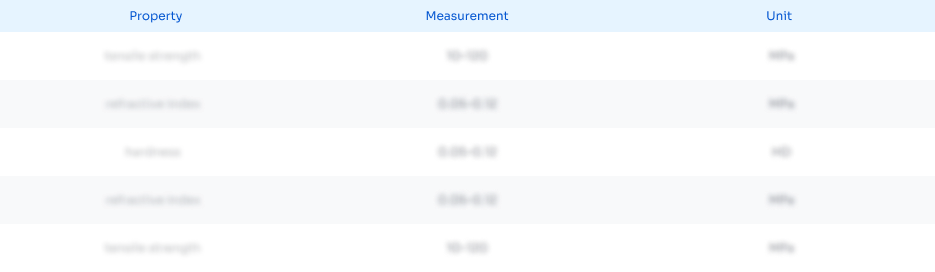
Abstract
Description
Claims
Application Information
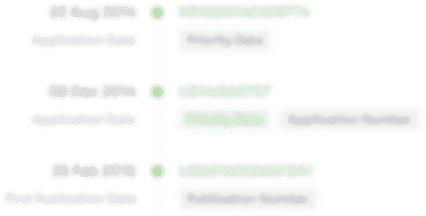
- R&D Engineer
- R&D Manager
- IP Professional
- Industry Leading Data Capabilities
- Powerful AI technology
- Patent DNA Extraction
Browse by: Latest US Patents, China's latest patents, Technical Efficacy Thesaurus, Application Domain, Technology Topic, Popular Technical Reports.
© 2024 PatSnap. All rights reserved.Legal|Privacy policy|Modern Slavery Act Transparency Statement|Sitemap|About US| Contact US: help@patsnap.com