Support vector regression method for simultaneously optimizing mean square error and square multiple correlation coefficient
A support vector regression and multiple correlation coefficient technology, applied in the computer field, can solve problems such as far-reaching influence, multiple setting parameters, and different influences, and achieve the effect of solving adjustments
Pending Publication Date: 2021-07-09
HOHAI UNIV
View PDF0 Cites 0 Cited by
- Summary
- Abstract
- Description
- Claims
- Application Information
AI Technical Summary
Problems solved by technology
However, there are many setting parameters. If you need to select a kernel function according to the feature number and capacity of the data set, after selecting the kernel function, you need to set the coefficient of the kernel function. Each kernel function has different coefficients, and these coefficients will bring about differences. The predicted results of the far-reaching
In addition, the influence of different coefficients on different targets is also different, especially the influence on the mean square error and squared complex correlation coefficient is rarely studied
[0003] Therefore, it is novel to take the prediction output of support vector regression: mean square error and squared complex correlation coefficient as the target at the same time, and use iterative algorithm to integrate it into support vector regression, so that the setting parameters of support vector regression can be optimized. research is lacking
Method used
the structure of the environmentally friendly knitted fabric provided by the present invention; figure 2 Flow chart of the yarn wrapping machine for environmentally friendly knitted fabrics and storage devices; image 3 Is the parameter map of the yarn covering machine
View moreImage
Smart Image Click on the blue labels to locate them in the text.
Smart ImageViewing Examples
Examples
Experimental program
Comparison scheme
Effect test
Embodiment 1
[0087] Take the Airfoil Self-Noise (airfoil self-noise data set) in the public data library of the University of California, Irvine as an example. The data download URL is http: / / Archive.ics.uci.edu / ml / datasets / Airfoil+Self- Noise, the data set capacity is 1503, and the number of features is 6.
the structure of the environmentally friendly knitted fabric provided by the present invention; figure 2 Flow chart of the yarn wrapping machine for environmentally friendly knitted fabrics and storage devices; image 3 Is the parameter map of the yarn covering machine
Login to View More PUM
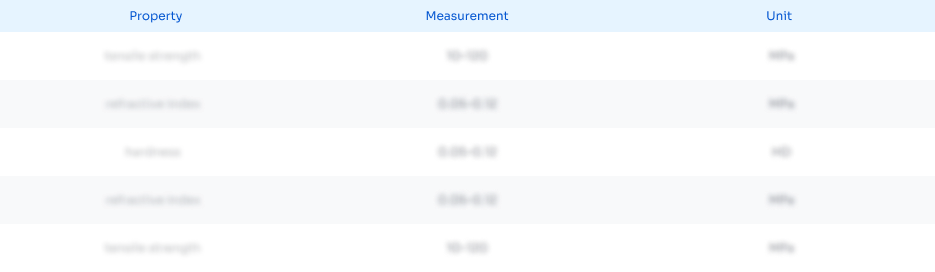
Abstract
The invention discloses a support vector regression method for simultaneously optimizing a mean square error and a square multiple correlation coefficient. The support vector regression method comprises the following specific steps: S1, segmenting and normalizing a data set; S2, initializing a support vector regression setting parameter group; S3, taking a mean square error and a square multiple correlation coefficient as multiple targets, and continuously updating a support vector regression setting parameter group; and S4, outputting optimized support vector regression setting parameters and corresponding mean square error and square multiple correlation coefficients. According to the method, the mean square error and the square multiple correlation coefficient are taken as multiple targets, the set parameters of support vector regression are optimized by using an iteration method, the mean square error is close to 0 as far as possible, and the square multiple correlation coefficient is close to 1 as far as possible, so that the problem of adjusting the set parameters of support vector regression is solved.
Description
technical field [0001] The invention relates to a support vector regression method for simultaneous optimization of mean square error and square complex correlation coefficient, belonging to the technical field of computers. Background technique [0002] Support vector regression is often used to build predictive models and is used in many application scenarios. However, there are many setting parameters. If you need to select a kernel function according to the feature number and capacity of the data set, after selecting the kernel function, you need to set the coefficient of the kernel function. Each kernel function has different coefficients, and these coefficients will bring about differences. The prediction results have a far-reaching impact. In addition, the impact of different coefficients on different targets is also different, especially the impact on the mean square error and squared complex correlation coefficient is rarely studied. [0003] Therefore, it is nove...
Claims
the structure of the environmentally friendly knitted fabric provided by the present invention; figure 2 Flow chart of the yarn wrapping machine for environmentally friendly knitted fabrics and storage devices; image 3 Is the parameter map of the yarn covering machine
Login to View More Application Information
Patent Timeline
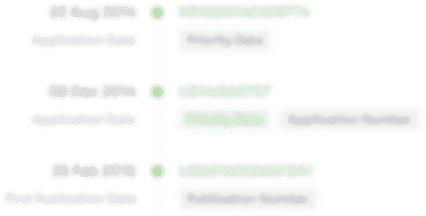
Patent Type & Authority Applications(China)
IPC IPC(8): G06K9/62G06N3/04
CPCG06N3/048G06F18/2414G06F18/214
Inventor 曹卫东欧阳骋姚志伟梁新利李力泓
Owner HOHAI UNIV
Who we serve
- R&D Engineer
- R&D Manager
- IP Professional
Why Patsnap Eureka
- Industry Leading Data Capabilities
- Powerful AI technology
- Patent DNA Extraction
Social media
Patsnap Eureka Blog
Learn More Browse by: Latest US Patents, China's latest patents, Technical Efficacy Thesaurus, Application Domain, Technology Topic, Popular Technical Reports.
© 2024 PatSnap. All rights reserved.Legal|Privacy policy|Modern Slavery Act Transparency Statement|Sitemap|About US| Contact US: help@patsnap.com