Micro-expression recognition method for representative AU region extraction based on multi-task learning
A multi-task learning and region extraction technology, applied in character and pattern recognition, instruments, computing, etc., can solve problems such as imbalance, large amount of calculation, easy overfitting of micro-expressions, and achieve the effect of increasing the number and improving performance
- Summary
- Abstract
- Description
- Claims
- Application Information
AI Technical Summary
Problems solved by technology
Method used
Image
Examples
Embodiment 1
[0122] A micro-expression recognition method for extracting representative AU regions based on multi-task learning, comprising the following steps:
[0123] A, the micro-expression video is preprocessed to obtain an image sequence comprising a face area and 68 key feature points thereof;
[0124] B. According to the 68 key feature points, obtain the position of the AU area, extract the optical flow characteristics in the AU area, set the number of representative AU areas, and obtain the most representative AU area;
[0125] C, data set division, according to the independent K-fold cross-validation method of the subject, the image sequence comprising the face region obtained in step A is divided into a training set and a test set to obtain a micro-expression training set and a micro-expression test set;
[0126] D. Send the face image sequence processed in step A into the AU mask feature extraction network model, calculate pixel-based cross-entropy loss and dice loss, and train...
Embodiment 2
[0132] According to the micro-expression recognition method for extracting a representative AU region based on multi-task learning described in Embodiment 1, the difference is that:
[0133] In step A, the micro-expression video is preprocessed, including framing, face key feature point detection, face cropping, TIM interpolation, and face scaling;
[0134] 1) Framing: divide the micro-expression video into micro-expression image sequences according to the frame rate of the micro-expression video;
[0135] 2) Face key feature point detection: use the Dlib vision library to detect 68 key feature points of the micro-expression image sequence; such as eyes, nose tip, mouth corner points, eyebrows, and contour points of various parts of the face. The detection effect is as follows: figure 2 shown;
[0136] 3) Face cropping: determine the position of the face frame according to the 68 key feature points of the positioned person;
[0137] In the horizontal direction, the midpoint ...
PUM
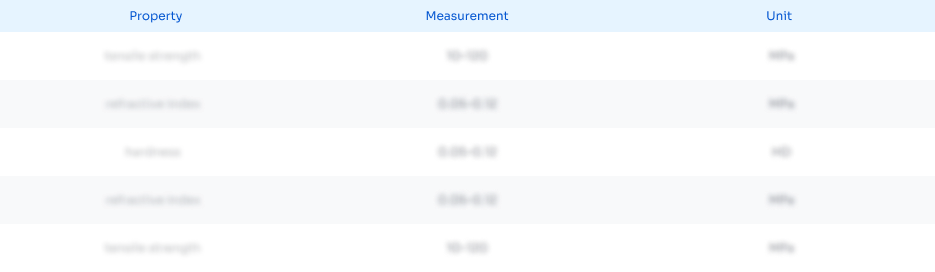
Abstract
Description
Claims
Application Information
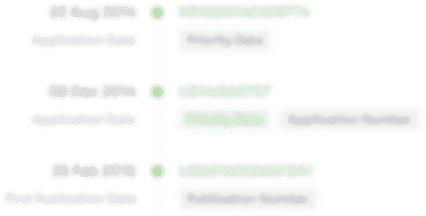
- R&D Engineer
- R&D Manager
- IP Professional
- Industry Leading Data Capabilities
- Powerful AI technology
- Patent DNA Extraction
Browse by: Latest US Patents, China's latest patents, Technical Efficacy Thesaurus, Application Domain, Technology Topic.
© 2024 PatSnap. All rights reserved.Legal|Privacy policy|Modern Slavery Act Transparency Statement|Sitemap