Space-time combination prediction method based on CNN-LSTM and deep learning
A combination of forecasting and deep learning technology, applied in neural learning methods, power generation forecasting and forecasting in AC networks, can solve the problems of not considering the potential space-time connection of wind farms, the limitations of forecasting accuracy, etc., and improve the forecasting accuracy and stability, the effects of good predictive performance
- Summary
- Abstract
- Description
- Claims
- Application Information
AI Technical Summary
Problems solved by technology
Method used
Image
Examples
Embodiment Construction
[0076] In order to facilitate those skilled in the art to better understand the present invention, the present invention will be described in further detail below in conjunction with the accompanying drawings and specific embodiments. The following is only exemplary and does not limit the protection scope of the present invention.
[0077] Such as figure 1 As shown, the embodiment of the present invention discloses a space-time combination prediction method based on CNN-LSTM and deep learning, comprising the following steps:
[0078] S1. Analyze the time and space correlation of the original data set through the MI mutual information algorithm, eliminate redundant information between the data, and reduce the dimension of the original data.
[0079] S2. Establish the MI-CNN-LSTM model to predict the data after dimension reduction in step S1, wherein the CNN network is used to extract the spatial information of each site, and the LSTM network is used to obtain dependency informa...
PUM
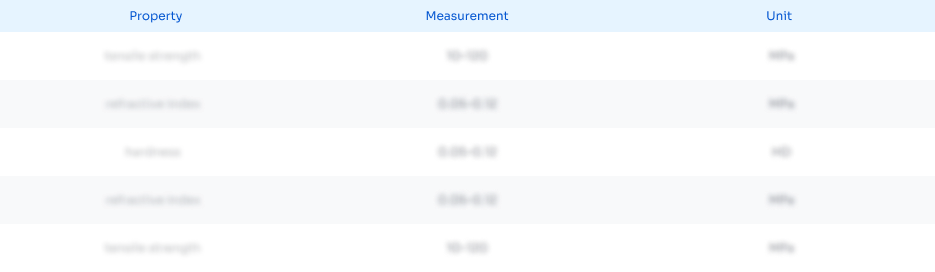
Abstract
Description
Claims
Application Information
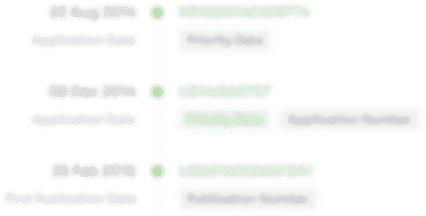
- R&D Engineer
- R&D Manager
- IP Professional
- Industry Leading Data Capabilities
- Powerful AI technology
- Patent DNA Extraction
Browse by: Latest US Patents, China's latest patents, Technical Efficacy Thesaurus, Application Domain, Technology Topic, Popular Technical Reports.
© 2024 PatSnap. All rights reserved.Legal|Privacy policy|Modern Slavery Act Transparency Statement|Sitemap|About US| Contact US: help@patsnap.com