Attention mechanism-based lightweight semantic segmentation model construction method
A semantic segmentation and construction method technology, applied in the field of image processing, can solve problems such as ignoring information, and achieve the effects of not being over-fitting, facilitating actual deployment, and improving performance
- Summary
- Abstract
- Description
- Claims
- Application Information
AI Technical Summary
Problems solved by technology
Method used
Image
Examples
Embodiment Construction
[0047] The following will clearly and completely describe the technical solutions in the embodiments of the present invention with reference to the accompanying drawings in the embodiments of the present invention. Obviously, the described embodiments are only some, not all, embodiments of the present invention. Based on the embodiments of the present invention, all other embodiments obtained by persons of ordinary skill in the art without making creative efforts belong to the protection scope of the present invention.
[0048] refer to figure 1 As shown, the present invention discloses a method for constructing a lightweight semantic segmentation model based on an attention mechanism, comprising the following steps:
[0049] Given an image I, the corresponding true label map GT constitutes the training set:
[0050] Step 1. Model establishment, using AHSP module, Channel Attention Sum (channel attention sum), Criss-Cross Attention Sum (cross attention sum), Channel Split (ch...
PUM
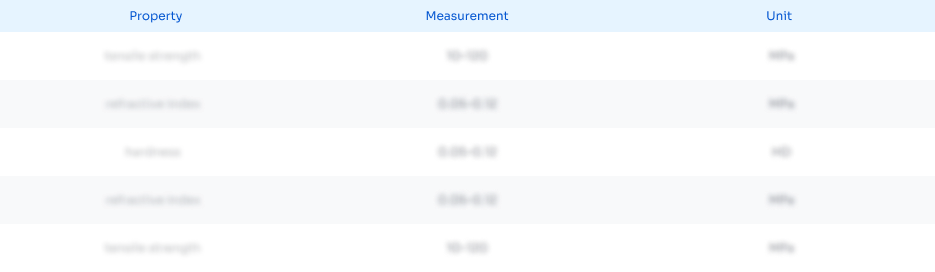
Abstract
Description
Claims
Application Information
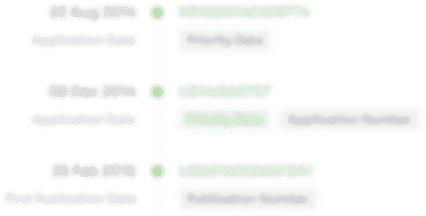
- R&D
- Intellectual Property
- Life Sciences
- Materials
- Tech Scout
- Unparalleled Data Quality
- Higher Quality Content
- 60% Fewer Hallucinations
Browse by: Latest US Patents, China's latest patents, Technical Efficacy Thesaurus, Application Domain, Technology Topic, Popular Technical Reports.
© 2025 PatSnap. All rights reserved.Legal|Privacy policy|Modern Slavery Act Transparency Statement|Sitemap|About US| Contact US: help@patsnap.com