A method for identifying long-tailed distributions with two branches and multiple centers
A branch and long-tail technology, applied in the field of double-branch and multi-center long-tail distribution recognition, to achieve good generalization ability and good recognition and classification effects
- Summary
- Abstract
- Description
- Claims
- Application Information
AI Technical Summary
Problems solved by technology
Method used
Image
Examples
Embodiment
[0044] This embodiment provides a method for long-tail distribution recognition with two branches and multiple centers, the flow chart of which is shown in figure 2 , wherein, the method of the present embodiment includes the following steps:
[0045] S1. Initialize two samplers, one adopts default sampling, and the picture obtained by the sampling is input into the default branch, and the other uses a resampling strategy for sampling, and the picture obtained by the sampling is input into the resampling branch.
[0046] Here, the default sampler samples each image with the same probability. Resampling sampling strategy. Before calculating the sampling probability of each picture, it is first necessary to make statistics on the training data set and calculate the number of pictures corresponding to each category. Here, the number of pictures owned by the i-th category is , remember that the number of pictures with the largest number is , the sum of pictures of all categor...
PUM
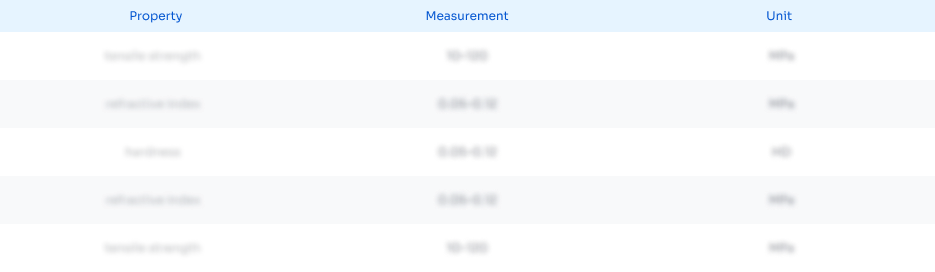
Abstract
Description
Claims
Application Information
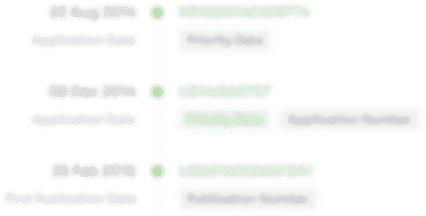
- R&D Engineer
- R&D Manager
- IP Professional
- Industry Leading Data Capabilities
- Powerful AI technology
- Patent DNA Extraction
Browse by: Latest US Patents, China's latest patents, Technical Efficacy Thesaurus, Application Domain, Technology Topic, Popular Technical Reports.
© 2024 PatSnap. All rights reserved.Legal|Privacy policy|Modern Slavery Act Transparency Statement|Sitemap|About US| Contact US: help@patsnap.com