Enterprise industry secondary industry multi-label classifier based on deep learning algorithm
A deep learning, multi-label technology, applied in special data processing applications, biological neural network models, structured data retrieval, etc., can solve the problems of low accuracy, difficult maintenance, troublesome and other problems, and improve the training time is too long. , the effect of improving the accuracy
- Summary
- Abstract
- Description
- Claims
- Application Information
AI Technical Summary
Problems solved by technology
Method used
Image
Examples
Embodiment 1
[0039] see figure 1 and figure 2 , the present invention provides a technical solution: a multi-label classifier for enterprise industry secondary industry based on deep learning algorithm, which consists of a collection module, a preprocessing module, a management module, a model building module, a training verification module, an input module, and a display module. consists of:
[0040] The collection module is used to collect the business scope information of the enterprise;
[0041] The preprocessing module is used to preprocess the business scope information of the enterprise;
[0042] The management module is used to manually index the business scope information of the enterprise, and to produce training sets, validation sets and test sets for multi-label classification training;
[0043] The model building module is used to build the Albert+TextCNN model using the training set;
[0044] The training and verification module is used to train the established Albert+Te...
Embodiment 2
[0070] see figure 1 and figure 2 , the present invention provides a technical solution: a multi-label classifier for enterprise industry secondary industry based on deep learning algorithm, which consists of a collection module, a preprocessing module, a management module, a model building module, a training verification module, an input module, and a display module. consists of:
[0071] The collection module is used to collect the business scope information of the enterprise;
[0072] The preprocessing module is used to preprocess the business scope information of the enterprise;
[0073] The management module is used to manually index the business scope information of the enterprise, and to produce training sets, validation sets and test sets for multi-label classification training;
[0074] The model building module is used to build the Albert+TextCNN model using the training set;
[0075] The training and verification module is used to train the established Albert+Te...
PUM
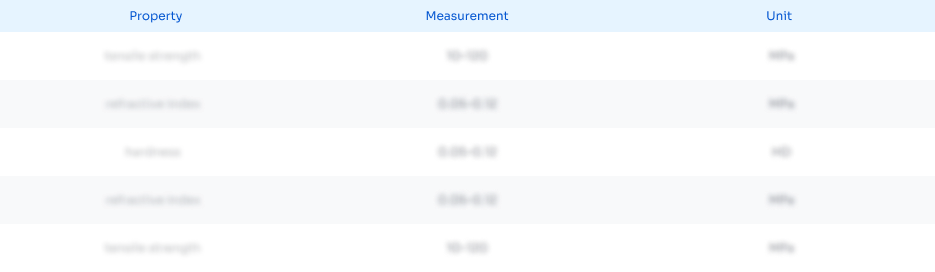
Abstract
Description
Claims
Application Information
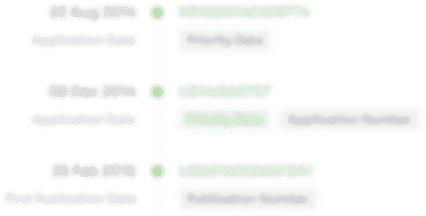
- R&D Engineer
- R&D Manager
- IP Professional
- Industry Leading Data Capabilities
- Powerful AI technology
- Patent DNA Extraction
Browse by: Latest US Patents, China's latest patents, Technical Efficacy Thesaurus, Application Domain, Technology Topic, Popular Technical Reports.
© 2024 PatSnap. All rights reserved.Legal|Privacy policy|Modern Slavery Act Transparency Statement|Sitemap|About US| Contact US: help@patsnap.com