Adaptive updating method applied to time sequence LSTM parameter prediction model
A forecasting model and time series technology, applied in forecasting, data processing applications, biological neural network models, etc., can solve problems such as the average forecast error exceeding the expected value and the decline of model forecasting accuracy.
- Summary
- Abstract
- Description
- Claims
- Application Information
AI Technical Summary
Problems solved by technology
Method used
Image
Examples
Embodiment Construction
[0034] The invention designs an adaptive update method applied to the time series LSTM parameter prediction model, provides an update strategy for the automatic update of the prediction model, not only can use the standby prediction model to update the prediction model in time, but also can use the dynamic The adjustment coefficient improves the prediction accuracy of the parameters of the prediction model. The flowchart of the adaptive update method of the LSTM parameter prediction model is as follows figure 1 The specific operation steps are as follows:
[0035] Step 1: Use the parameter sample data (X 1 , X 2 ,...X num ) as the modeling data, find the mean of the modeling data As shown in formula (1);
[0036]
[0037] Step 2: Normalize the modeling data, as shown in formula (2) and formula (3), where is the mean of the modeling data described in step 1, S is the standard deviation, and the normalized data is used as the modeling data num is the amount of model...
PUM
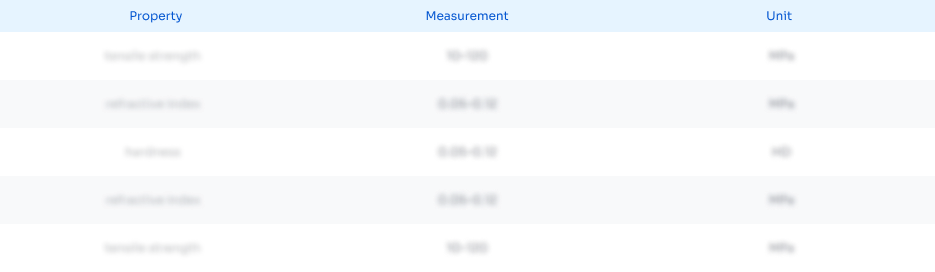
Abstract
Description
Claims
Application Information
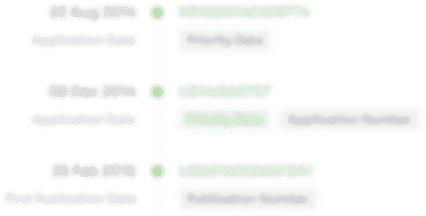
- R&D
- Intellectual Property
- Life Sciences
- Materials
- Tech Scout
- Unparalleled Data Quality
- Higher Quality Content
- 60% Fewer Hallucinations
Browse by: Latest US Patents, China's latest patents, Technical Efficacy Thesaurus, Application Domain, Technology Topic, Popular Technical Reports.
© 2025 PatSnap. All rights reserved.Legal|Privacy policy|Modern Slavery Act Transparency Statement|Sitemap|About US| Contact US: help@patsnap.com