Incomplete Multi-View Clustering Method Based on Missing Graph Reconstruction and Adaptive Nearest Neighbors
A clustering method and multi-view technology, applied in the computer field, can solve problems such as inability to learn missing data consistency features, inability to handle missing view scenarios, inability to learn multi-view data consistency graph structure, etc.
- Summary
- Abstract
- Description
- Claims
- Application Information
AI Technical Summary
Problems solved by technology
Method used
Image
Examples
Embodiment
[0109] The following is the implementation process of this embodiment:
[0110] 1, as figure 1 and figure 2 As shown, the incomplete multi-view is first processed into and format, where represents the data observed on the vth view, Represents the correspondence between the observation data on the vth view and the incomplete multi-view data.
[0111] 2, get the data and After that, the Euclidean distance is used to calculate the distance between the observed data on each view, and the missing graph structure A on each view is obtained accordingly. v . details as follows:
[0112] c1. The Euclidean distance is used to calculate the distance between the observed data on each view, namely in represents the i-th observation data on the v-th view, ||·|| F represents the Frobenius norm of the matrix;
[0113] c2. Calculate the neighbor graph structure of the observed data on each view by the following formula:
[0114]
[0115] in, represent data At the...
PUM
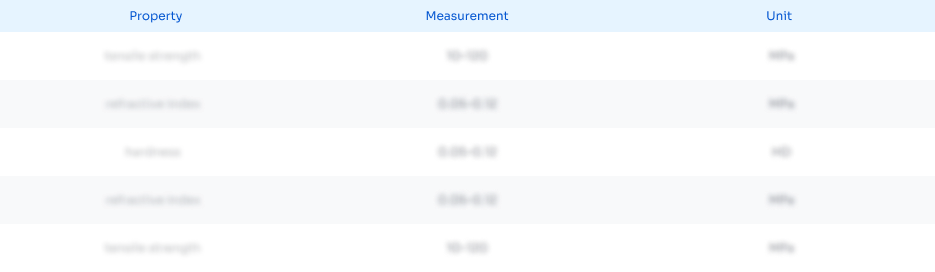
Abstract
Description
Claims
Application Information
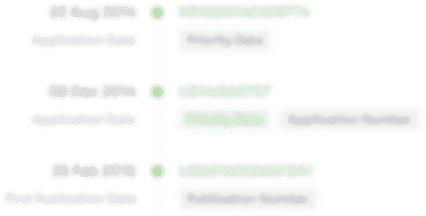
- R&D
- Intellectual Property
- Life Sciences
- Materials
- Tech Scout
- Unparalleled Data Quality
- Higher Quality Content
- 60% Fewer Hallucinations
Browse by: Latest US Patents, China's latest patents, Technical Efficacy Thesaurus, Application Domain, Technology Topic, Popular Technical Reports.
© 2025 PatSnap. All rights reserved.Legal|Privacy policy|Modern Slavery Act Transparency Statement|Sitemap|About US| Contact US: help@patsnap.com