SNN workload prediction method and system
A workload and prediction method technology, applied in the field of spiking neural networks, can solve problems such as poor applicability of random connectivity networks, low communication load prediction accuracy, and deeper understanding of SNN workloads, etc., to ensure high-performance operation Effect
- Summary
- Abstract
- Description
- Claims
- Application Information
AI Technical Summary
Problems solved by technology
Method used
Image
Examples
Embodiment Construction
[0052] The present invention will be further described below in conjunction with the accompanying drawings and specific embodiments, so that those skilled in the art can better understand the present invention and implement it, but the examples given are not intended to limit the present invention.
[0053] like figure 1 Shown, be the SNN workload prediction method in the preferred embodiment of the present invention, it comprises the following steps:
[0054] S1, build SNN workload model based on NEST emulator, described SNN workload model comprises: memory load model, computation load model and communication load model; Specifically:
[0055] In the memory load model, the total memory consumption M of each process includes M 0 , M n and M s Three parts, as shown in the following formula:
[0056]
[0057] Among them, M 0 is the basic memory consumption required for NEST to run, neuron memory overhead M n and the synaptic memory overhead M s As shown in the followin...
PUM
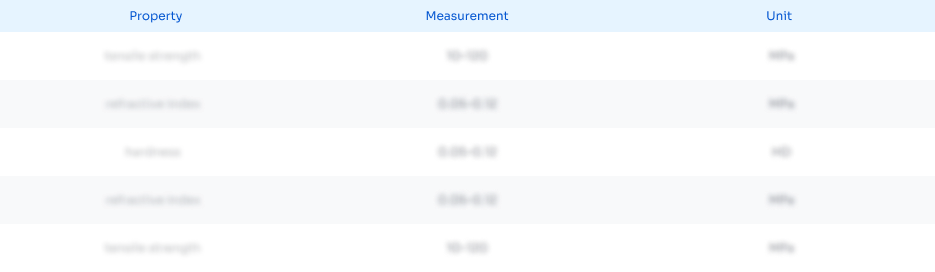
Abstract
Description
Claims
Application Information
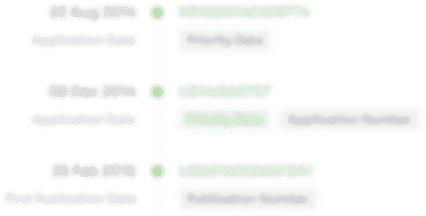
- R&D Engineer
- R&D Manager
- IP Professional
- Industry Leading Data Capabilities
- Powerful AI technology
- Patent DNA Extraction
Browse by: Latest US Patents, China's latest patents, Technical Efficacy Thesaurus, Application Domain, Technology Topic.
© 2024 PatSnap. All rights reserved.Legal|Privacy policy|Modern Slavery Act Transparency Statement|Sitemap