Physically realizable laser radar 3D point cloud confrontation sample generation method and system
A technology against samples and lidar, applied in instruments, computing models, machine learning, etc., can solve the problems of not considering the principle of lidar collection point cloud information, deceiving 3D point cloud target detectors, etc., to reduce the false prediction rate Effect
- Summary
- Abstract
- Description
- Claims
- Application Information
AI Technical Summary
Problems solved by technology
Method used
Image
Examples
Embodiment Construction
[0026] The embodiments of the present invention will be described in detail below in conjunction with the accompanying drawings. This embodiment is based on the technical solution of the present invention, and provides detailed implementation methods and specific operating procedures, but the scope of protection of the present invention is not limited to the following embodiments. .
[0027] Taking the application scenario of self-driving cars as an example, deploy lidar and 3D point cloud target detectors on the self-driving car. The laser radar uses infrared laser to measure the distance to the surrounding obstacles, and the point cloud information is expressed in the form of coordinates and intensity. Store it as the original 3D point cloud data. Subsequently, the 3D point cloud target detector takes the original 3D point cloud data as input, and then makes a decision to judge the target in front or around.
[0028] Such as figure 1 As shown, a physically realizable LiDAR...
PUM
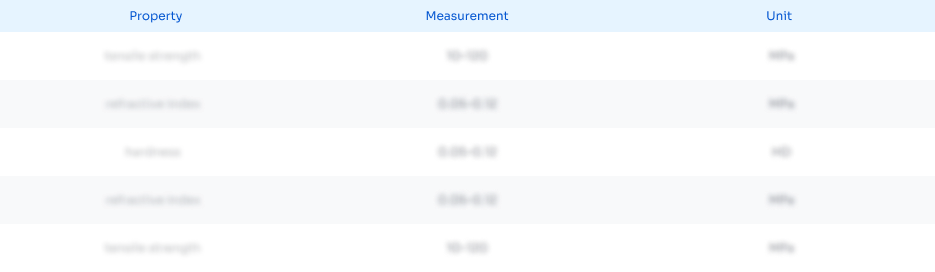
Abstract
Description
Claims
Application Information
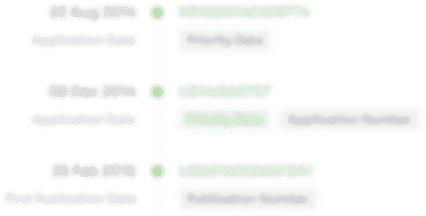
- R&D Engineer
- R&D Manager
- IP Professional
- Industry Leading Data Capabilities
- Powerful AI technology
- Patent DNA Extraction
Browse by: Latest US Patents, China's latest patents, Technical Efficacy Thesaurus, Application Domain, Technology Topic, Popular Technical Reports.
© 2024 PatSnap. All rights reserved.Legal|Privacy policy|Modern Slavery Act Transparency Statement|Sitemap|About US| Contact US: help@patsnap.com