Mobile phone surface defect accurate grading method based on mixed attention deformation convolutional neural network
A convolutional neural network and classification method technology, applied in the field of solid waste treatment, can solve the problems of increasing the difficulty of model recognition, the impact of image clarity, and poor recognition accuracy, so as to improve the overall recognition performance, reduce the objective impact, The effect of restoring clarity
- Summary
- Abstract
- Description
- Claims
- Application Information
AI Technical Summary
Problems solved by technology
Method used
Image
Examples
Embodiment Construction
[0062] The present invention adopts the following technical solutions and implementation steps:
[0063] 2. A method for accurate classification of surface defects of used mobile phones based on mixed attention mechanism deformation convolutional neural network. By designing a mixed attention mechanism model to optimize the performance of the model, a recognition model based on deformation convolutional neural network is established to realize the surface defects of used mobile phones. Accurate grading of defects, including the following steps:
[0064] (1) Data collection of waste mobile phones
[0065] The degree of damage to the screen of the used mobile phone seriously affects the recycling price of the used mobile phone, so it is an essential step to accurately classify the surface defects of the used mobile phone; first, by shooting the screen of the used mobile phone, upload the captured image to the computer connected to the industrial camera Finally, use the software...
PUM
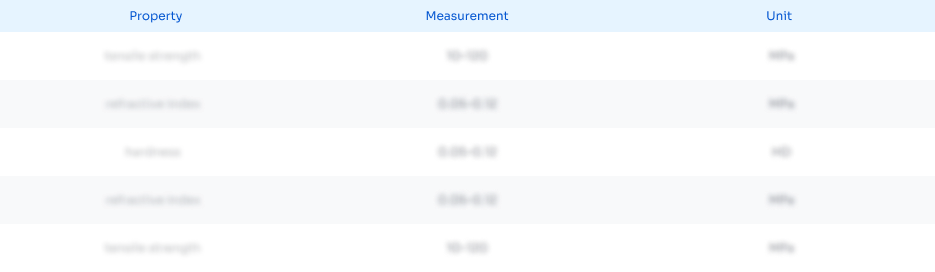
Abstract
Description
Claims
Application Information
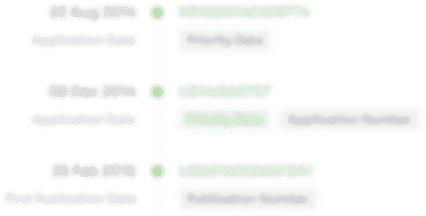
- R&D
- Intellectual Property
- Life Sciences
- Materials
- Tech Scout
- Unparalleled Data Quality
- Higher Quality Content
- 60% Fewer Hallucinations
Browse by: Latest US Patents, China's latest patents, Technical Efficacy Thesaurus, Application Domain, Technology Topic, Popular Technical Reports.
© 2025 PatSnap. All rights reserved.Legal|Privacy policy|Modern Slavery Act Transparency Statement|Sitemap|About US| Contact US: help@patsnap.com