Sample-equalizable crankshaft surface defect detection method and detection system
A defect detection and crankshaft technology, applied in image analysis, instruments, calculations, etc., can solve problems such as the imbalance of positive and negative samples, the impact of algorithm performance, etc., and achieve the effect of sample expansion
- Summary
- Abstract
- Description
- Claims
- Application Information
AI Technical Summary
Problems solved by technology
Method used
Examples
Embodiment 1
[0026] In a first aspect, the present invention provides a method for detecting surface defects of crankshafts with equal samples, comprising the following steps:
[0027] S1. Constructing a training sample data set, including: applying magnetic powder to the surface of the crankshaft and irradiating it with fluorescence to obtain a surface image of the crankshaft; judging and marking the state of the surface image to obtain normal image data , image data with defects one, define x image as a data sample, only when x When all the images are normal images, the x Only this sample composed of images is marked as a positive sample; x As long as there is a defective image in the image, the x This sample composed of images is marked as a negative sample; the positive samples and negative samples constitute a training sample data set; among them, x The specific value of is given by , simplified . That is, randomly selected from the normal image data N x composition of im...
Embodiment 2
[0035] In a second aspect, the present invention provides a crankshaft surface defect detection system with equalized samples, including a magnetic powder application device, a fluorescence irradiation device, a photographing device, and a deep learning neural network identification module;
[0036] The magnetic powder applying device is used for applying magnetic powder to the surface of the crankshaft.
[0037] The fluorescent irradiation device is used to perform fluorescent irradiation on the surface of the crankshaft, so as to judge the surface state of the crankshaft.
[0038] The photographing device is used for acquiring the surface image data of the crankshaft and corresponding to the corresponding surface state, and obtaining a training sample data set based on this; wherein, obtaining the training sample data set specifically includes: the acquired crankshaft surface image includes: normal image data , image data with defects one, define x image as a data sampl...
PUM
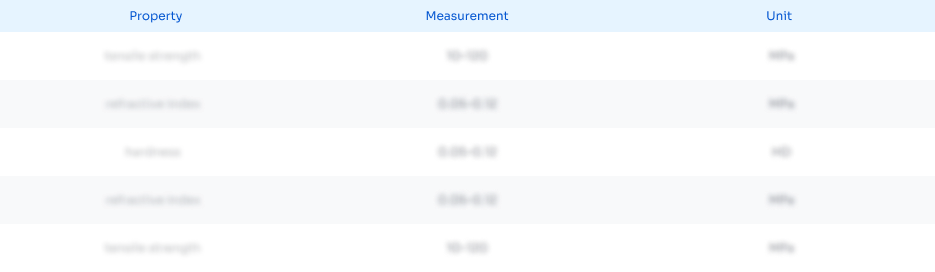
Abstract
Description
Claims
Application Information
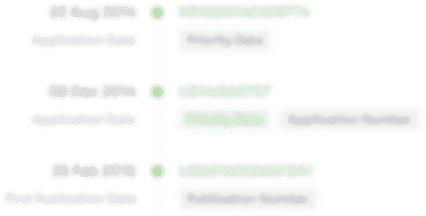
- R&D Engineer
- R&D Manager
- IP Professional
- Industry Leading Data Capabilities
- Powerful AI technology
- Patent DNA Extraction
Browse by: Latest US Patents, China's latest patents, Technical Efficacy Thesaurus, Application Domain, Technology Topic, Popular Technical Reports.
© 2024 PatSnap. All rights reserved.Legal|Privacy policy|Modern Slavery Act Transparency Statement|Sitemap|About US| Contact US: help@patsnap.com