Dangerous vehicle identification method based on cross attention mechanism dynamic knowledge propagation
A dangerous vehicle and identification method technology, which is applied in the field of dangerous vehicle identification based on the dynamic knowledge dissemination of the cross-attention mechanism, can solve the problem of high calculation volume, achieve the effect of improving detection performance, solving calculation volume and accuracy problems
- Summary
- Abstract
- Description
- Claims
- Application Information
AI Technical Summary
Problems solved by technology
Method used
Image
Examples
Embodiment 1
[0060] This embodiment introduces a method for identifying a dangerous vehicle, including:
[0061] Obtain the image frame of the dangerous vehicle to be identified;
[0062] Input the obtained image frames into the pre-trained dangerous vehicle recognition model;
[0063] According to the output of the dangerous vehicle identification model, determine whether there is a dangerous vehicle that makes a dangerous action in the image, and if so, output the dangerous vehicle information;
[0064] Among them, refer to figure 1 As shown, the dangerous vehicle recognition model includes a teacher network and a student network, the teacher network includes a first feature extraction network and a first temporal feature fusion network, and the student network includes a second feature extraction network, a second temporal feature fusion network, and a dynamic knowledge dissemination network. and prediction network; the first feature extraction network and the second feature extractio...
Embodiment 2
[0112] This embodiment introduces a dangerous vehicle identification device, including:
[0113] an image sampling module configured to acquire image frames of the dangerous vehicle to be identified;
[0114] an action classification module configured to input the acquired image frames into a pre-trained dangerous vehicle identification model;
[0115] an identification result output module, configured to determine whether there is a dangerous vehicle making dangerous actions in the image according to the output of the dangerous vehicle identification model, and if so, output dangerous vehicle information;
[0116] The dangerous vehicle identification model includes a teacher network and a student network, the teacher network includes a first feature extraction network and a first temporal feature fusion network, and the student network includes a second feature extraction network, a second temporal feature fusion network, and a dynamic knowledge dissemination network. networ...
Embodiment 3
[0125] This embodiment introduces a computer-readable storage medium on which a computer program is stored. When the computer program is executed by a processor, the method for identifying a dangerous vehicle as described in Embodiment 1 is implemented.
[0126] To sum up the above embodiments, the experiments show that the method of using the teacher model for reasoning in the present invention is effective for video recognition, and greatly improves the accuracy and robustness of dangerous vehicle recognition. And the complexity is low, the realization is simple, and the calculation speed is relatively fast.
[0127] As will be appreciated by those skilled in the art, the embodiments of the present application may be provided as a method, a system, or a computer program product. Accordingly, the present application may take the form of an entirely hardware embodiment, an entirely software embodiment, or an embodiment combining software and hardware aspects. Furthermore, the...
PUM
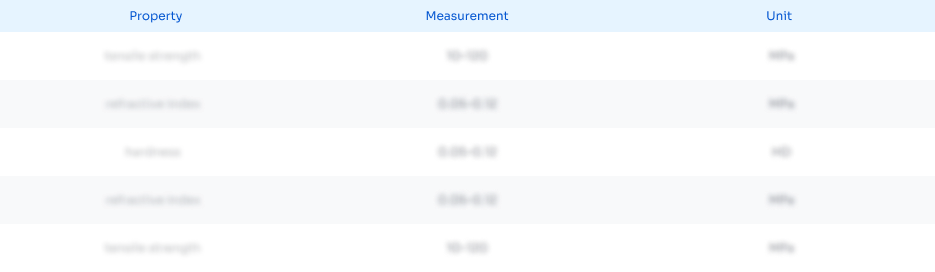
Abstract
Description
Claims
Application Information
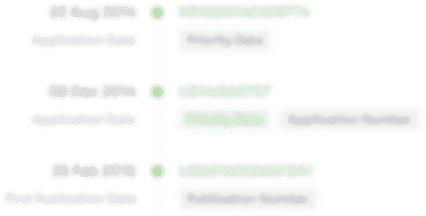
- R&D
- Intellectual Property
- Life Sciences
- Materials
- Tech Scout
- Unparalleled Data Quality
- Higher Quality Content
- 60% Fewer Hallucinations
Browse by: Latest US Patents, China's latest patents, Technical Efficacy Thesaurus, Application Domain, Technology Topic, Popular Technical Reports.
© 2025 PatSnap. All rights reserved.Legal|Privacy policy|Modern Slavery Act Transparency Statement|Sitemap|About US| Contact US: help@patsnap.com