Controlling a non-linear process
a non-linear process and control technology, applied in the field of prediction modeling and control, can solve the problems of inability to arrive at analytical solutions in most real-world systems, inability to accurately estimate and inability to apply each of these two approaches to real-world complex systems. to achieve the effect of improving the estimate of the current state of the process
- Summary
- Abstract
- Description
- Claims
- Application Information
AI Technical Summary
Benefits of technology
Problems solved by technology
Method used
Image
Examples
Embodiment Construction
Incorporation by Reference
[0078]The following references are hereby incorporated by reference in their entirety as though fully and completely set forth herein:
[0079]U.S. application Ser. No. 10 / 842,157, titled “PARAMETRIC UNIVERSAL NONLINEAR DYNAMICS APPROXIMATOR AND USE”, filed May. 10, 2004;
[0080]U.S. patent application Ser. No. 10 / 350,830, titled “Parameterizing a Steady State Model Using Derivative Constraints”, filed Jan. 24, 2003, whose inventor was Gregory D. Martin.
Terms
[0081]The following is a glossary of terms used in the present application:
[0082]Objective Function—a mathematical expression of a desired behavior or goal.
[0083]Constraint—a limitation on a property or attribute used to limit the search space in an optimization process.
[0084]Optimizer—a tool or process that operates to determine an optimal set of parameter values for a system or process by solving an objective function, optionally subject to one or more constraints.
[0085]Control Variables—process outputs, e...
PUM
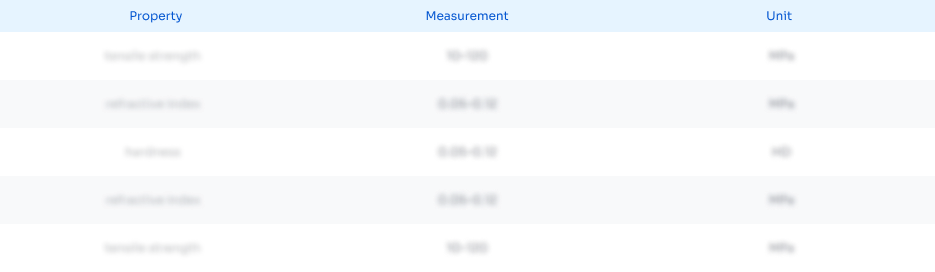
Abstract
Description
Claims
Application Information
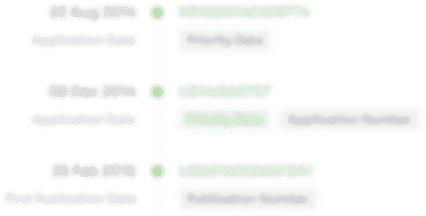
- R&D
- Intellectual Property
- Life Sciences
- Materials
- Tech Scout
- Unparalleled Data Quality
- Higher Quality Content
- 60% Fewer Hallucinations
Browse by: Latest US Patents, China's latest patents, Technical Efficacy Thesaurus, Application Domain, Technology Topic, Popular Technical Reports.
© 2025 PatSnap. All rights reserved.Legal|Privacy policy|Modern Slavery Act Transparency Statement|Sitemap|About US| Contact US: help@patsnap.com