Data discretization method based on category-attribute relation dependency
A discretized and dependent technology, applied in electrical digital data processing, special data processing applications, instruments, etc., can solve problems such as information loss, unreasonableness, and impact on machine learning accuracy, and achieve the effect of reducing inconsistency and high precision.
- Summary
- Abstract
- Description
- Claims
- Application Information
AI Technical Summary
Problems solved by technology
Method used
Image
Examples
Embodiment Construction
[0048] The specific process of the Improved CAIM program is as follows:
[0049] Input: A dataset with m instances, t decision classes and s conditional attributes.
[0050] The first stage:
[0051] (1) Calculate the difference set of each attribute, and sort the attributes in order of importance from small to large a 1 , a 2 ,...a s (a 1 Represents the least important attribute, a s represents the most important attribute)
[0052] (2)For(a i =a 1 ;i<=s;i++)
[0053] {
[0054] Step1:
[0055] find attribute a i The minimum value in x min and the maximum value x max ;
[0056] attribute a i All the different values in are arranged in ascending order {x min , x 2 ,...x max};
[0057] Calculate the intermediate value between all adjacent different values as a candidate breakpoint, the calculation formula is
[0058] d i = x i + ...
PUM
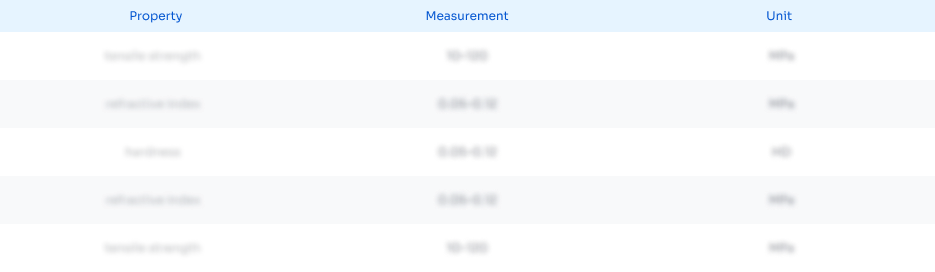
Abstract
Description
Claims
Application Information
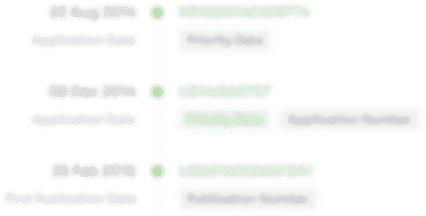
- Generate Ideas
- Intellectual Property
- Life Sciences
- Materials
- Tech Scout
- Unparalleled Data Quality
- Higher Quality Content
- 60% Fewer Hallucinations
Browse by: Latest US Patents, China's latest patents, Technical Efficacy Thesaurus, Application Domain, Technology Topic, Popular Technical Reports.
© 2025 PatSnap. All rights reserved.Legal|Privacy policy|Modern Slavery Act Transparency Statement|Sitemap|About US| Contact US: help@patsnap.com