Method for realizing classification of scene images
A technology of scene image and classification method, which is applied in the direction of instruments, character and pattern recognition, computer parts, etc., can solve the problem of not being able to effectively use various feature information of images, scene image classification has not yet achieved satisfactory results, and ignoring classifiers Complementary advantages of different features and other issues to achieve the effect of improving classification accuracy, fast computing speed, and good noise resistance
Active Publication Date: 2010-08-25
INST OF AUTOMATION CHINESE ACAD OF SCI
View PDF1 Cites 41 Cited by
- Summary
- Abstract
- Description
- Claims
- Application Information
AI Technical Summary
Problems solved by technology
The existing scene image classification methods often use a single classifier, and only select a single-stage classifier to complete the final classification according to the empirical observation of the scene image. This method cannot effectively use various feature information of the image, and ignores the The fusion and cascading of classifiers can take advantage of the complementary advantages of different features, so that the classification of scene images has not yet achieved satisfactory results
Method used
the structure of the environmentally friendly knitted fabric provided by the present invention; figure 2 Flow chart of the yarn wrapping machine for environmentally friendly knitted fabrics and storage devices; image 3 Is the parameter map of the yarn covering machine
View moreImage
Smart Image Click on the blue labels to locate them in the text.
Smart ImageViewing Examples
Examples
Experimental program
Comparison scheme
Effect test
Embodiment Construction
the structure of the environmentally friendly knitted fabric provided by the present invention; figure 2 Flow chart of the yarn wrapping machine for environmentally friendly knitted fabrics and storage devices; image 3 Is the parameter map of the yarn covering machine
Login to View More PUM
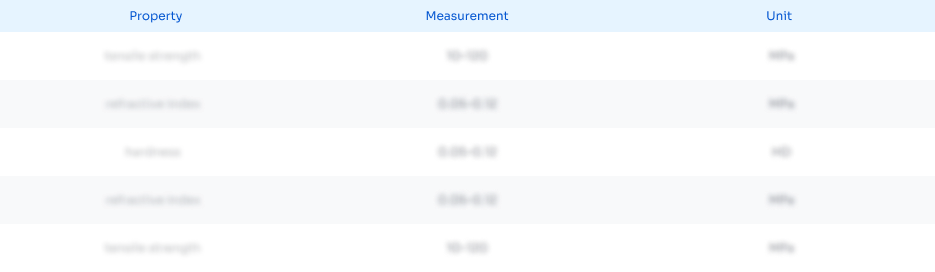
Abstract
The invention discloses a method for realizing classification of scene images. In the method, the scene images are classified by adopting two stages of classifiers, wherein the first-stage classifier obtains candidate classifications by utilizing global structural information characteristics, and judges similar classification pairs according to classification results; and the second-stage classifier distinguishes similar classifications by utilizing local texture information characteristics. By adopting the cascade of the classifiers and comprehensively utilizing the global structural information characteristics and the local texture information characteristics of the scene images, the method can realize robust classification of different scene classifications and effectively distinguish similar scene classifications.
Description
technical field The invention belongs to the technical field of pattern recognition and information processing, and relates to the automatic processing technology of digital images, in particular to a classification method of scene images. Background technique With the wide application of various image acquisition devices such as digital cameras, cameras, ultra-high-speed scanners, and the rapid development of the Internet, the number of digital images has grown exponentially. According to incomplete statistics, more than 18 billion digital images were generated in 2004 alone. images, and Google Image Search has indexed hundreds of millions of images, so how to effectively classify images is becoming more and more important. A person can usually recognize more than 10,000 categories of visual objects, and the recognition process is fast and effortless. It is also robust to perspective, light, occlusion and background confusion, and it only takes a few minutes to recognize a ...
Claims
the structure of the environmentally friendly knitted fabric provided by the present invention; figure 2 Flow chart of the yarn wrapping machine for environmentally friendly knitted fabrics and storage devices; image 3 Is the parameter map of the yarn covering machine
Login to View More Application Information
Patent Timeline
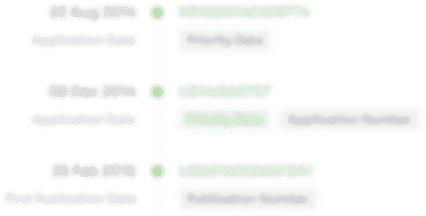
IPC IPC(8): G06K9/66
Inventor 王春恒程刚肖柏华李心洁
Owner INST OF AUTOMATION CHINESE ACAD OF SCI
Features
- R&D
- Intellectual Property
- Life Sciences
- Materials
- Tech Scout
Why Patsnap Eureka
- Unparalleled Data Quality
- Higher Quality Content
- 60% Fewer Hallucinations
Social media
Patsnap Eureka Blog
Learn More Browse by: Latest US Patents, China's latest patents, Technical Efficacy Thesaurus, Application Domain, Technology Topic, Popular Technical Reports.
© 2025 PatSnap. All rights reserved.Legal|Privacy policy|Modern Slavery Act Transparency Statement|Sitemap|About US| Contact US: help@patsnap.com