Sparse representation-based background clutter quantification method
A technology of background clutter and quantification method, which is applied in the field of image processing, can solve the problems of not considering the characteristics of human visual perception, violating the physical essence of background clutter, and the scale of clutter cannot be reasonably reflected, so as to achieve consistent target detection probability, Predict accurate results
- Summary
- Abstract
- Description
- Claims
- Application Information
AI Technical Summary
Problems solved by technology
Method used
Image
Examples
Embodiment Construction
[0028] Reference figure 1 The implementation steps of the background clutter quantization method based on sparse representation of the present invention are as follows:
[0029] Step 1. Take the pixel value of the target image as a unit, and form the target vector in the order of the initial column number from small to large
[0030] x={t 1,1 , T 2,1 , T 3,1 ,..., t C,1 , T 1,2 ,..., t C, D } T
[0031] Where t i, j Represents the target pixel value at (i, j), C and D represent the number of rows and columns of the target image, respectively, and T represents the transposition operation of the vector.
[0032] Step 2: Divide the background image to be quantized into N small units of equal size, and the size of each small unit in the horizontal direction and the vertical direction is twice the corresponding size of the target.
[0033] The size of N is determined by the size of the background image to be quantized A×B and the size of each small unit M=C×D, namely Among them, A and B ...
PUM
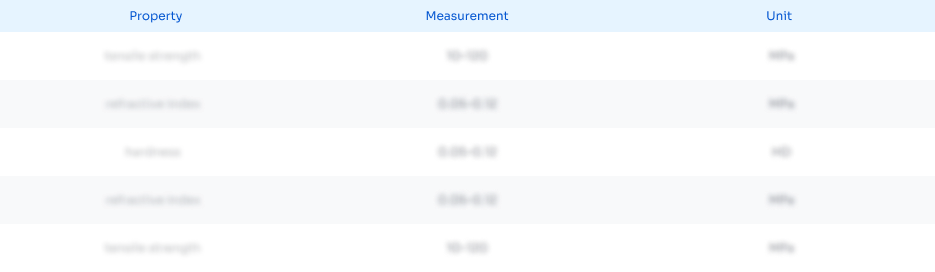
Abstract
Description
Claims
Application Information
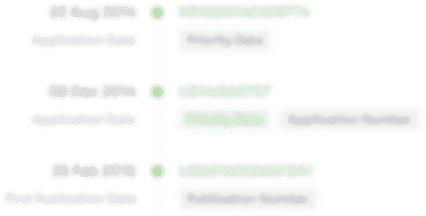
- R&D
- Intellectual Property
- Life Sciences
- Materials
- Tech Scout
- Unparalleled Data Quality
- Higher Quality Content
- 60% Fewer Hallucinations
Browse by: Latest US Patents, China's latest patents, Technical Efficacy Thesaurus, Application Domain, Technology Topic, Popular Technical Reports.
© 2025 PatSnap. All rights reserved.Legal|Privacy policy|Modern Slavery Act Transparency Statement|Sitemap|About US| Contact US: help@patsnap.com