Group concept-based improved Fast-Newman clustering method applied to complex network
A technology of complex network and clustering method, applied in the field of optimization class clustering based on group thinking to improve the objective function, can solve the problem that the network cluster structure cannot be completely and accurately described, and achieve the effect of outstanding clustering effect.
- Summary
- Abstract
- Description
- Claims
- Application Information
AI Technical Summary
Problems solved by technology
Method used
Image
Examples
Embodiment Construction
[0031] The method of the present invention will be described below in conjunction with the accompanying drawings and specific embodiments.
[0032] As the network scale gradually expands, the probability that nodes in the network have global information gradually decreases. In large-scale complex networks, nodes only have global information with extremely small probability; usually, nodes have local information centered on themselves. In the clustering algorithm, using the knowledge and strategies in the global environment, although the theoretical global optimal solution can be found, the most realistic cluster structure cannot be obtained. Therefore, it is necessary to obtain the real network cluster structure by simulating the range of local information mastered by nodes and the decision-making environment when they are clustered, and searching for the optimal clustering result under this environment.
[0033] The invention proposes and defines the concept of "group" based o...
PUM
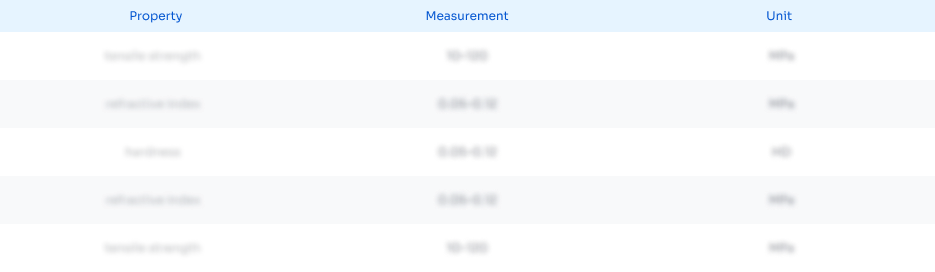
Abstract
Description
Claims
Application Information
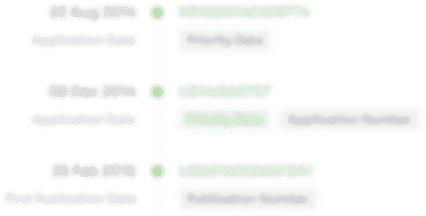
- R&D
- Intellectual Property
- Life Sciences
- Materials
- Tech Scout
- Unparalleled Data Quality
- Higher Quality Content
- 60% Fewer Hallucinations
Browse by: Latest US Patents, China's latest patents, Technical Efficacy Thesaurus, Application Domain, Technology Topic, Popular Technical Reports.
© 2025 PatSnap. All rights reserved.Legal|Privacy policy|Modern Slavery Act Transparency Statement|Sitemap|About US| Contact US: help@patsnap.com