Image fusion method based on multi-scale dictionary learning
A multi-scale dictionary and image fusion technology, applied in the field of image fusion, can solve the problem of not being able to analyze data at multiple scales
- Summary
- Abstract
- Description
- Claims
- Application Information
AI Technical Summary
Problems solved by technology
Method used
Image
Examples
example 1
[0063] Example 1. Fusion example of non-defaced image
[0064] In this example, infrared visible image pairs and remote sensing image pairs are fused separately, and there is no standard fusion result. The implementation steps of the multi-scale dictionary learning and fusion process in this example are as follows:
[0065] Multi-scale dictionary learning is shown below :
[0066] (1) Decompose each training image with 3-level db4 wavelet transform, and the training data adopts the source fusion image itself;
[0067] (2) Initialize all sub-dictionaries D b ∈R 64×256 ,b=1,2,...,10(3×3+1);
[0068] (3) For all subbands, the sliding window with a step size of 1 and a size of 8×8 extracts blocks in order from upper left to lower right, then straightens the blocks and arranges them in turn to form a matrix, and each subband is arranged as a matrix;
[0069] (4) Use the K-SVD algorithm to learn a sub-dictionary D for each matrix b , the K-SVD algorithm allows the error ε t...
example 2
[0086] Example 2. Fusion example of noisy image
[0087] In practical applications, source images containing noise are often obtained, so how to suppress the influence of noise on the fusion result in the process of fused images is also an important aspect that needs to be considered in the current fusion method. In the past, the denoising and fusion processing of most image fusion methods were carried out separately, but in recent years, image fusion methods based on sparse representation have shown excellent performance in completing denoising while merging, so in this example, the present invention is further described The fusion method's ability to suppress noise.
[0088] The image in this example is a multi-focus image with standard fusion results. Figure 4(a) and (b) are noise-free near-focus and far-focus images, and (c) is the standard fusion result, both of which are 256×256 in size. Add Gaussian white noise with mean value 0 and variance σ(σ=5,10,15,20,25) to Figur...
example 3
[0095] Example 3. Generalization example of dictionary
[0096] The examples in the previous two sections discussed the fusion results of several methods in unstained images and noise images. It is worth noting that the training data of the dictionary comes from the source image itself. In fact, since each atom of the dictionary represents a structural prototype of the source image, the dictionary trained for one type of image can be directly applied to another set of images of the same type, so that there is no need to retrain the dictionary during fusion. Based on this First, this subsection discusses the generalization ability of dictionaries in image fusion.
[0097] The multi-scale dictionary learning part of this example does not re-learn the multi-scale dictionary, but directly uses the multi-scale dictionary of remote sensing images trained in Example 1 for the fusion of the other two sets of remote sensing images; the steps and parameter settings of the fusion process...
PUM
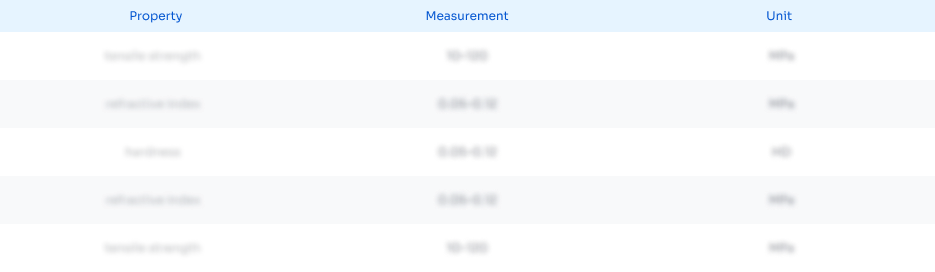
Abstract
Description
Claims
Application Information
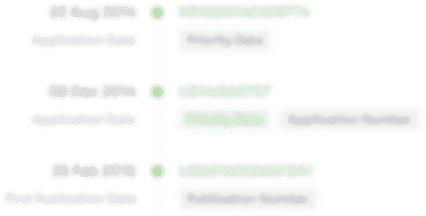
- R&D Engineer
- R&D Manager
- IP Professional
- Industry Leading Data Capabilities
- Powerful AI technology
- Patent DNA Extraction
Browse by: Latest US Patents, China's latest patents, Technical Efficacy Thesaurus, Application Domain, Technology Topic.
© 2024 PatSnap. All rights reserved.Legal|Privacy policy|Modern Slavery Act Transparency Statement|Sitemap