Hidden structure learning-based image digest generation method
A technology of image summarization and abstract, applied in the direction of editing/combining graphics or text, special data processing applications, instruments, etc., can solve the problems of information differences, failure to comprehensively consider complementary information and redundant information, subject differences, etc., and achieve good results. effect, high information coverage, low information redundancy effect
- Summary
- Abstract
- Description
- Claims
- Application Information
AI Technical Summary
Problems solved by technology
Method used
Image
Examples
Embodiment
[0071] 19 picture collections are selected from the database, and different picture collections are associated with different themes, for example, plane crash, briefcase, sea, factory workers, etc. The picture collection of each topic contains 30 to 70 pictures, and 6 pictures are manually selected as the abstract picture collection. First, the color histogram, visual word and direction gradient histogram features are extracted from these pictures, and then the three features are normalized and fused, so that each picture is projected into a 2450-dimensional feature space. In the process of normalization, the normalization method of normalizing to 0.1 to 0.9 is used. For example, in the color histogram features of all pictures, the maximum value is max and the minimum value is min, then for a value x of a certain dimension, the normalized feature value will become 0.1+(x-min)(max- min) × (0.9-0.1). In this way, the minimum value of the color histogram feature is converted to...
PUM
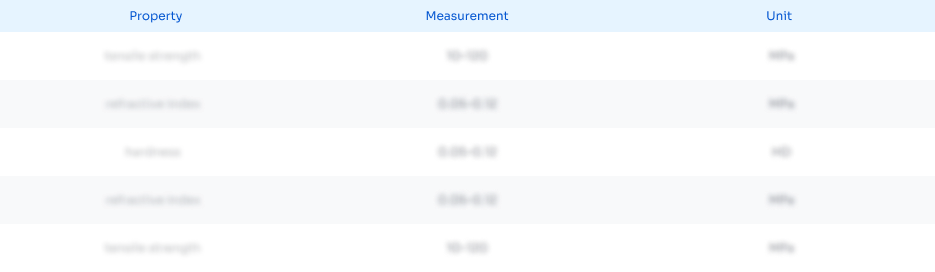
Abstract
Description
Claims
Application Information
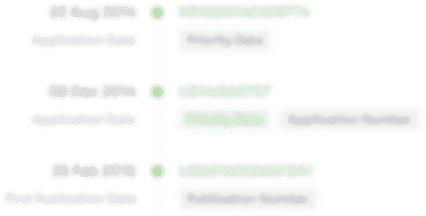
- R&D Engineer
- R&D Manager
- IP Professional
- Industry Leading Data Capabilities
- Powerful AI technology
- Patent DNA Extraction
Browse by: Latest US Patents, China's latest patents, Technical Efficacy Thesaurus, Application Domain, Technology Topic, Popular Technical Reports.
© 2024 PatSnap. All rights reserved.Legal|Privacy policy|Modern Slavery Act Transparency Statement|Sitemap|About US| Contact US: help@patsnap.com