Meta learning-based combined prediction method for time-varying nonlinear load of electrical power system
A nonlinear load and combined forecasting technology, applied in forecasting, instrumentation, data processing applications, etc., can solve problems such as limited applicability of mathematical models, large forecast deviations, and inability to predict model estimation and adjustment.
- Summary
- Abstract
- Description
- Claims
- Application Information
AI Technical Summary
Problems solved by technology
Method used
Image
Examples
Embodiment Construction
[0047] The present invention will be described in further detail below in conjunction with the accompanying drawings and embodiments. However, the invention is not limited to the examples given.
[0048] according to figure 1 The forecast process adopts the primary curve model, the quadratic curve model, the cubic curve model and the simple exponential curve model to predict the load of a total of 2880 points in a regional power grid from March 1 to March 10, 2004. The prediction results are as follows Figure 5 shown. The prediction result is used as the input of the meta-prediction base predictor, the feature attributes of the prediction stage are extracted, and the input gating network is used to calculate the combination weight to obtain the combination prediction result. The structure of the combination predictor of meta-learning is as follows: figure 2 . According to the minimum energy function of the overall mean square error, cyclically adjust the parameters of the...
PUM
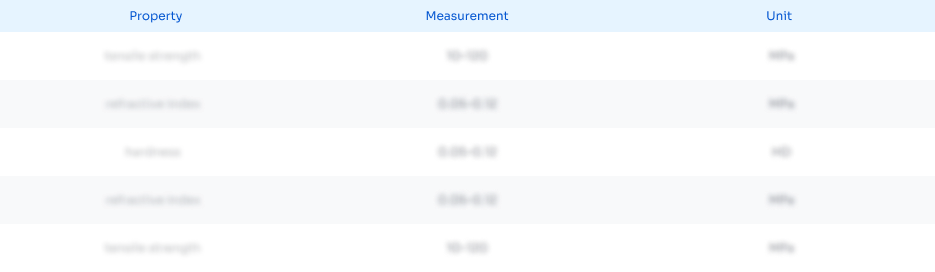
Abstract
Description
Claims
Application Information
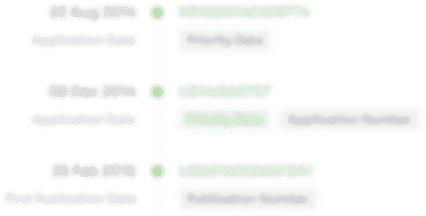
- R&D Engineer
- R&D Manager
- IP Professional
- Industry Leading Data Capabilities
- Powerful AI technology
- Patent DNA Extraction
Browse by: Latest US Patents, China's latest patents, Technical Efficacy Thesaurus, Application Domain, Technology Topic, Popular Technical Reports.
© 2024 PatSnap. All rights reserved.Legal|Privacy policy|Modern Slavery Act Transparency Statement|Sitemap|About US| Contact US: help@patsnap.com