Semi-supervised speech feature variable factor decomposition method
A voice feature, semi-supervised technology, applied in the direction of instruments, character and pattern recognition, computer parts, etc., can solve the problems of recognition rate influence, indistinguishability, confusion, etc., to avoid mutual interference and improve recognition accuracy.
- Summary
- Abstract
- Description
- Claims
- Application Information
AI Technical Summary
Problems solved by technology
Method used
Image
Examples
Embodiment Construction
[0015] figure 1 The general idea of the method of the present invention is given. First, the speech is preprocessed to obtain the spectrogram, and the spectrogram blocks of different sizes are input into the unsupervised feature learning network SAE, and the convolution kernels of different sizes are obtained through pre-training, and then after convolution , pooling operation to form a local invariant feature y. y is used as the input of the semi-supervised convolutional neural network, and y is decomposed into four types of features by minimizing four different loss function terms.
[0016] The preprocessed speech signal is divided into l i× h i Spectral blocks of different sizes, i represents the number of spectral blocks, different sizes of spectral blocks are input into the unsupervised feature learning network SAE, pre-trained to obtain convolution kernels of different sizes, and then use convolution kernels of different sizes to compare the entire language Convolve...
PUM
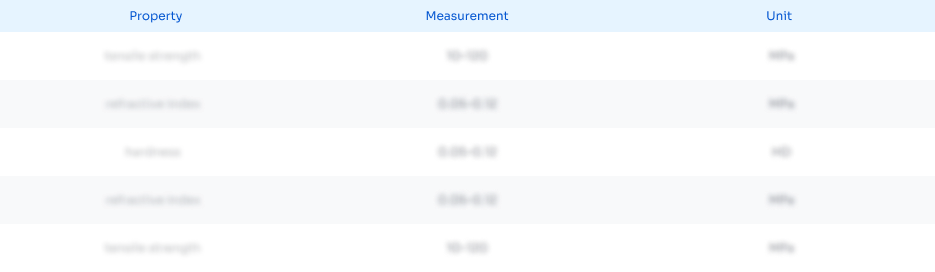
Abstract
Description
Claims
Application Information
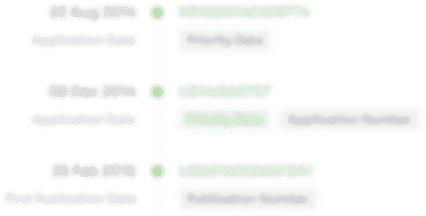
- R&D Engineer
- R&D Manager
- IP Professional
- Industry Leading Data Capabilities
- Powerful AI technology
- Patent DNA Extraction
Browse by: Latest US Patents, China's latest patents, Technical Efficacy Thesaurus, Application Domain, Technology Topic, Popular Technical Reports.
© 2024 PatSnap. All rights reserved.Legal|Privacy policy|Modern Slavery Act Transparency Statement|Sitemap|About US| Contact US: help@patsnap.com