Feature extraction and feature selection methods for background multi-source data
A feature selection method, multi-source data technology, applied in data processing applications, character and pattern recognition, instruments, etc., can solve problems such as the impact of prediction results
- Summary
- Abstract
- Description
- Claims
- Application Information
AI Technical Summary
Problems solved by technology
Method used
Image
Examples
Embodiment Construction
[0029] Such as figure 1 As shown, for background multi-source data, specific feature extraction methods are used for different source data. For the extracted multi-source data features, the Group Lasso method is used for group feature selection, and a machine learning model is further established on the selected group features. Predict off-grid users.
[0030] Such as figure 2 As shown, the training set and test set are divided for the data from May 2013 to February 2014.
[0031] Such as image 3 As shown in the figure, it is a line chart of the daily online time of 50 users in May. The amount of data about users going online and offline is huge and contains a lot of information.
[0032] Such as Figure 4 , Figure 5 As shown, the method for extracting online time trend features based on multi-scale histogram statistics proposed by the present invention includes the following steps:
[0033] (1) This time series is not a typical time series in the traditional sense, ...
PUM
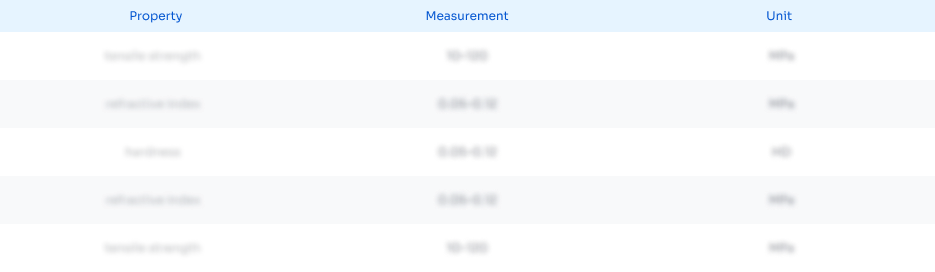
Abstract
Description
Claims
Application Information
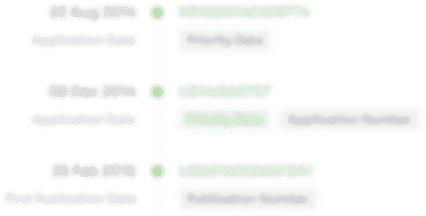
- R&D Engineer
- R&D Manager
- IP Professional
- Industry Leading Data Capabilities
- Powerful AI technology
- Patent DNA Extraction
Browse by: Latest US Patents, China's latest patents, Technical Efficacy Thesaurus, Application Domain, Technology Topic, Popular Technical Reports.
© 2024 PatSnap. All rights reserved.Legal|Privacy policy|Modern Slavery Act Transparency Statement|Sitemap|About US| Contact US: help@patsnap.com