Large-scale heterogeneous data oriented co-clustering method
A technology of heterogeneous data and clustering methods, applied in text database clustering/classification, structured data retrieval, unstructured text data retrieval, etc., can solve high time complexity, unbalanced, abnormally sparse relational data, etc. problem, to achieve fast joint clustering, improve accuracy, and reduce sparsity
- Summary
- Abstract
- Description
- Claims
- Application Information
AI Technical Summary
Problems solved by technology
Method used
Image
Examples
Embodiment Construction
[0041] The present invention will be described in further detail below in conjunction with the accompanying drawings.
[0042] When large-scale heterogeneous data is jointly clustered, the scale growth of different types of entities is unbalanced, and the heterogeneous relational data also becomes extremely sparse, resulting in imbalance and sparse problems. In view of the above two problems, the present invention proposes a heterogeneous relationship matrix joint clustering method based on the correlation matrix, and its overall schematic diagram is as follows figure 1 shown. It transforms the traditional non-negative matrix factorization problem into a two-stage factorization problem. Firstly, the association relationship corresponding to a class of entities with a smaller scale is extracted to construct an association matrix, and the partition indicator matrix is obtained through symmetric non-negative matrix decomposition. Compared with the original relationship matrix...
PUM
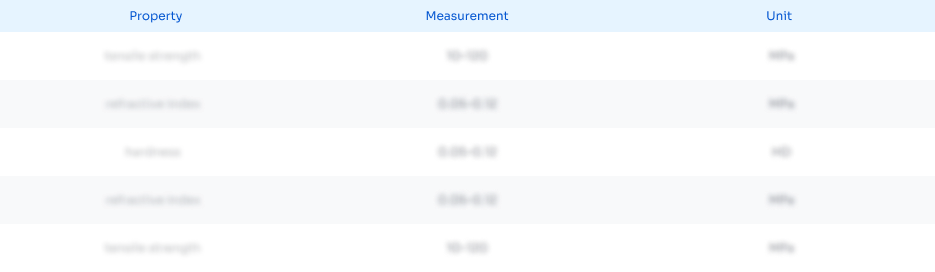
Abstract
Description
Claims
Application Information
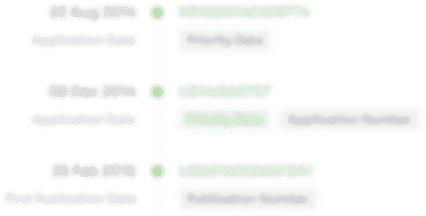
- R&D Engineer
- R&D Manager
- IP Professional
- Industry Leading Data Capabilities
- Powerful AI technology
- Patent DNA Extraction
Browse by: Latest US Patents, China's latest patents, Technical Efficacy Thesaurus, Application Domain, Technology Topic, Popular Technical Reports.
© 2024 PatSnap. All rights reserved.Legal|Privacy policy|Modern Slavery Act Transparency Statement|Sitemap|About US| Contact US: help@patsnap.com