Image characteristic extraction method, pedestrian detection method and device
An image feature extraction and pedestrian detection technology, applied in the field of image processing, can solve problems such as limited identification ability, and achieve the effect of rich information, high accuracy, and enhanced identification.
- Summary
- Abstract
- Description
- Claims
- Application Information
AI Technical Summary
Problems solved by technology
Method used
Image
Examples
Embodiment 1
[0052] This embodiment provides an image feature extraction method, such as figure 1 shown, including the following steps:
[0053] S1. Acquire mid-image features in the target image.
[0054] Among them, the middle-level features refer to the image histogram features constructed based on visual keywords and bag-of-words models. Generally, the underlying local features (such as SIFT) are extracted first, and then clustered to obtain a visual keyword dictionary. Finally, based on these visual Keywords quantify the original underlying local features and obtain them in the form of bag-of-words histograms. Compared with commonly used image bottom-level features, also known as basic features (such as color, gradient), middle-level features can represent richer patterns and information, and also provide a basis for obtaining higher-level semantic information.
[0055] S2. Obtain initial values of hidden semantic features.
[0056] In this embodiment, the hidden semantic feature...
Embodiment 2
[0080] This embodiment provides a specific implementation manner of an image feature extraction method, including the following process.
[0081] In the first step, basic features are extracted for each sample.
[0082] For each sample, local feature descriptors are first extracted at fixed step intervals, and then the k-means clustering algorithm is used to quantify these feature descriptors into a fixed-dimensional histogram based on the bag-of-words model as the basic feature of the sample. Specifically, it can be described as: given a dictionary of visual features Where M is the dimension of the dictionary, and w is the visual keyword in the dictionary, then each sample can be expressed as a histogram based on the visual word bag model {freq(w i ): i=1, 2, ..., M}, where freq(w i ) is the visual keyword w i frequency in each sample.
[0083] The second step is to extract hidden semantic features.
[0084] The extraction of hidden semantic features is a specific spars...
Embodiment 3
[0108] This embodiment provides a method for pedestrian detection, applying the image feature extraction method in Embodiment 1 to pedestrian detection, such as figure 2 shown, including the following steps:
[0109] S11. Extract basic features for each training sample. It is the same as that in Embodiment 2 and will not be repeated here.
[0110] S12. Obtain the implicit semantic feature of the basic feature, the extraction method is the same as that in Embodiments 1 and 2, which will not be repeated here.
[0111] S13. Establish a pedestrian detection model according to the hidden semantic features.
[0112] After obtaining the hidden semantic features X of the training samples, in this scheme, these hidden semantic features are counted in the form of histograms in the regular image units in a manner similar to the HOG feature. Specifically, for each non-zero x i , using the bilinear interpolation method to convert its absolute value|x i |Assigned to one of the four ce...
PUM
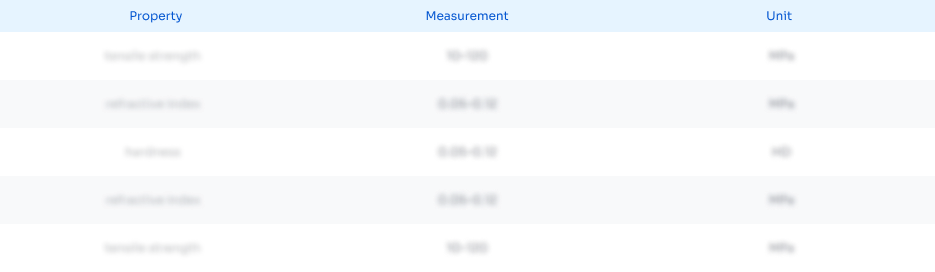
Abstract
Description
Claims
Application Information
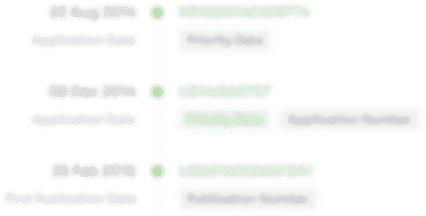
- Generate Ideas
- Intellectual Property
- Life Sciences
- Materials
- Tech Scout
- Unparalleled Data Quality
- Higher Quality Content
- 60% Fewer Hallucinations
Browse by: Latest US Patents, China's latest patents, Technical Efficacy Thesaurus, Application Domain, Technology Topic, Popular Technical Reports.
© 2025 PatSnap. All rights reserved.Legal|Privacy policy|Modern Slavery Act Transparency Statement|Sitemap|About US| Contact US: help@patsnap.com