Mapreduced short period load prediction method of multinucleated function learning SVM realizing multi-source heterogeneous data fusion
A multi-source heterogeneous data, short-term load forecasting technology, applied in forecasting, data processing applications, instruments, etc., can solve problems such as inability to accurately process and apply, and inability to meet short-term load forecasting accuracy and speed requirements.
- Summary
- Abstract
- Description
- Claims
- Application Information
AI Technical Summary
Problems solved by technology
Method used
Image
Examples
Embodiment Construction
[0039] In order to make the present invention more obvious and understandable, a detailed description is given below with preferred implementation examples and accompanying drawings.
[0040] Step 1. Configure the load forecasting platform: select the master node server and slave node computers, build a cluster distributed Hadoop platform, and configure the cluster environment JDK, SSH, HDFS, Mapreduce, etc.;
[0041] Step 2. Investigate the load situation of the distribution network area to be predicted: Investigate the load types of the underlying lines of the distribution network area to be predicted, such as 10KV dedicated lines, 35KV dedicated lines, and the composition of industrial, agricultural, commercial, and residential loads within the distribution network area. proportion;
[0042] Step 3. Select the types of multi-source heterogeneous data: According to the survey results of step 2, select M types of random multi-source heterogeneous factor eigenvalue attributes that af...
PUM
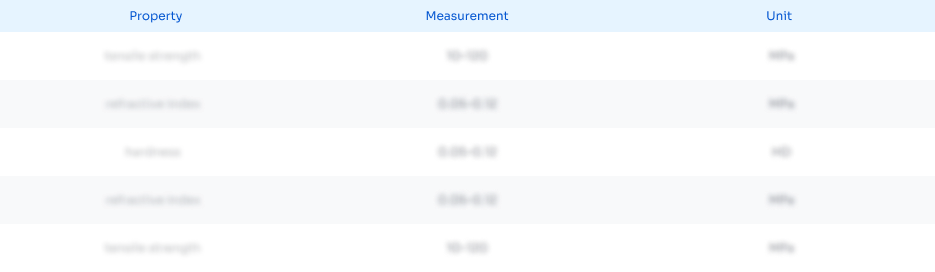
Abstract
Description
Claims
Application Information
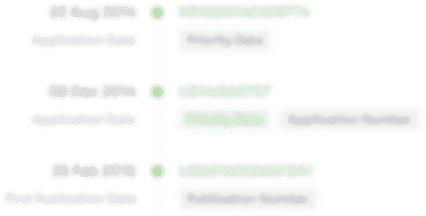
- R&D
- Intellectual Property
- Life Sciences
- Materials
- Tech Scout
- Unparalleled Data Quality
- Higher Quality Content
- 60% Fewer Hallucinations
Browse by: Latest US Patents, China's latest patents, Technical Efficacy Thesaurus, Application Domain, Technology Topic, Popular Technical Reports.
© 2025 PatSnap. All rights reserved.Legal|Privacy policy|Modern Slavery Act Transparency Statement|Sitemap|About US| Contact US: help@patsnap.com