Optimal Feature Subset Selection Method Based on Structural Vector Complementary of Classification Ability
A technology with classification ability and optimal features, applied in instruments, character and pattern recognition, computer parts, etc., and can solve problems such as small scores
- Summary
- Abstract
- Description
- Claims
- Application Information
AI Technical Summary
Problems solved by technology
Method used
Image
Examples
Embodiment 1
[0049] 1. Read the classification problem dataset.
[0050] Usually a classification problem dataset is a two-dimensional matrix, e.g. having features kind sample classification problem datasets such as figure 1 shown, where Indicates the first of the sample The eigenvalues of a feature, Indicates the first category of samples. Table 1 shows the expression values of some characteristic genes of some samples in the breast cancer data set, where the second row is the sample category, the third row is the expression value of the first feature on each sample, and so on for other rows, and one column represents a A sample is the expression value and category of each feature of a certain person. Read all the eigenvalues of each sample in the dataset into a two-dimensional array , read the category of each sample into a one-dimensional array middle.
[0051] Table 1 Expression values of some characteristic genes of some samples in the breast cancer dat...
Embodiment 2
[0096] Experimental result and data of the present invention:
[0097] The experimental data set of the present invention - breast cancer (breast), was downloaded from http: / / www.ccbm.jhu.edu / in 2007, see references. The breast dataset contains 5 categories, 9216 features and 54 samples. The traditional objective evaluation index is used to test the performance of the algorithm, which mainly includes the number of selected features and the accuracy of classification prediction. The number of features selected refers to the number of features selected by the feature selection method, and the accuracy of classification prediction is the The accuracy rate obtained by selecting a subset of features as input to the classifier. In order to verify the effectiveness of the method proposed in the present invention, it is compared with existing attribute selection methods such as FCBF, CFS, mrMr, and Relief. Because the mrMr and Relief methods only evaluate features and give sorting ...
PUM
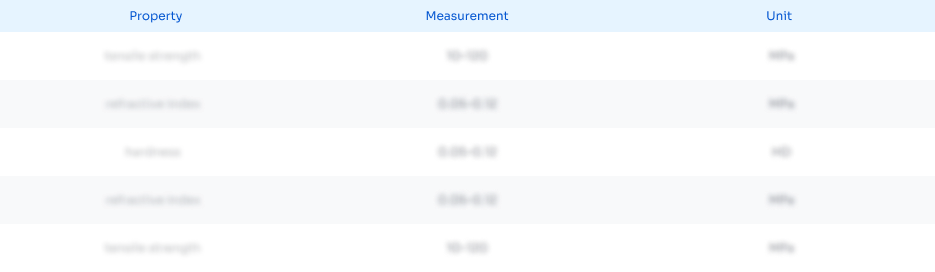
Abstract
Description
Claims
Application Information
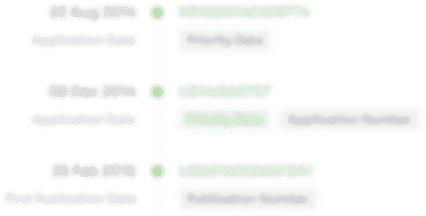
- R&D Engineer
- R&D Manager
- IP Professional
- Industry Leading Data Capabilities
- Powerful AI technology
- Patent DNA Extraction
Browse by: Latest US Patents, China's latest patents, Technical Efficacy Thesaurus, Application Domain, Technology Topic.
© 2024 PatSnap. All rights reserved.Legal|Privacy policy|Modern Slavery Act Transparency Statement|Sitemap