Feature selection method for high-dimensional data
A feature selection method and high-dimensional data technology, applied in the direction of instruments, calculations, character and pattern recognition, etc., can solve the problems that the feature dimension cannot be well determined, the calculation cost is high, and the accuracy rate is difficult to obtain, so as to achieve stable features Selection process, effect of good feature dimension
- Summary
- Abstract
- Description
- Claims
- Application Information
AI Technical Summary
Problems solved by technology
Method used
Image
Examples
Embodiment Construction
[0036] The following embodiments will further illustrate the present invention in conjunction with the accompanying drawings.
[0037] 1) The stability score of the feature:
[0038] Randomized Logistic Regression (RLR) is a stable selection technique that calculates a stable score for the features of the dataset by sampling multiple times. The present invention only needs to perform a scoring calculation on the data set to obtain a feature score, and does not need to perform repeated evaluation on the feature subsequently. Then a subset of features can be searched based on the different scores between features. The specific feature scoring results are as follows: figure 2 , which means that the higher the score corresponding to the feature, the stronger the importance of the feature.
[0039] 2) Selection of feature subsets:
[0040] Different features have different scores, and there are differences in scores between features. Sort the features in descending order of i...
PUM
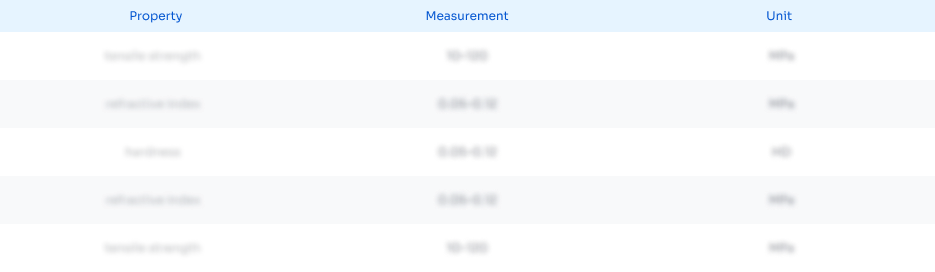
Abstract
Description
Claims
Application Information
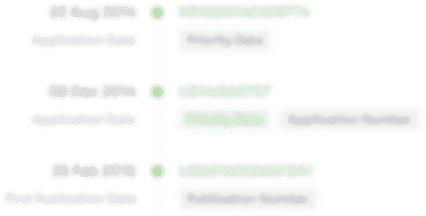
- R&D
- Intellectual Property
- Life Sciences
- Materials
- Tech Scout
- Unparalleled Data Quality
- Higher Quality Content
- 60% Fewer Hallucinations
Browse by: Latest US Patents, China's latest patents, Technical Efficacy Thesaurus, Application Domain, Technology Topic, Popular Technical Reports.
© 2025 PatSnap. All rights reserved.Legal|Privacy policy|Modern Slavery Act Transparency Statement|Sitemap|About US| Contact US: help@patsnap.com