Hyperspectral data dimensionality-reduction method based on sparse and low-rank representation graph
A low-rank representation and data dimensionality reduction technology, applied in the field of hyperspectral data dimensionality reduction, can solve problems such as lack of global constraints and loss of global data characteristics
- Summary
- Abstract
- Description
- Claims
- Application Information
AI Technical Summary
Problems solved by technology
Method used
Image
Examples
Embodiment Construction
[0043] The basic process of the present invention is as figure 1 As shown, it specifically includes the following steps:
[0044] 1) Input the hyperspectral data into the computer, and normalize the data.
[0045] Read the entire hyperspectral image, and use (min is the minimum value in each band, and max is the maximum value in each band) The normalization formula normalizes the entire data set.
[0046] 2) Select part of the data as training samples.
[0047] For the hyperspectral data that has been normalized, a certain amount of data is randomly selected for each category as training samples.
[0048] 3) Construction of sparse and low-rank representation graphs.
[0049] Sort the training samples so that the training samples of the same category are arranged together. Calculate the sparse low-rank representation graph W of each type of training samples according to formula (5) (l) , and compose W in the form of diagonal matrix blocks to generate a graph of all train...
PUM
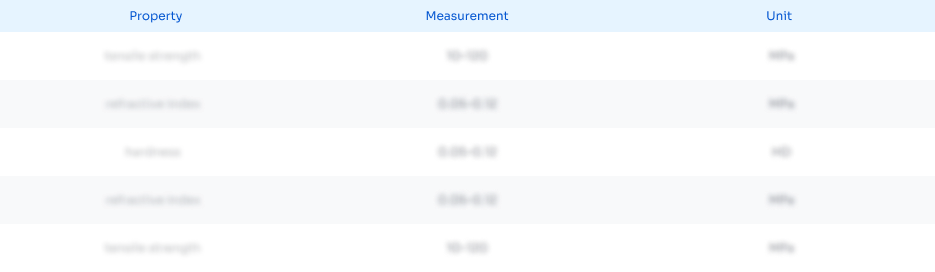
Abstract
Description
Claims
Application Information
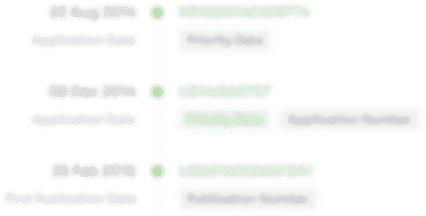
- R&D
- Intellectual Property
- Life Sciences
- Materials
- Tech Scout
- Unparalleled Data Quality
- Higher Quality Content
- 60% Fewer Hallucinations
Browse by: Latest US Patents, China's latest patents, Technical Efficacy Thesaurus, Application Domain, Technology Topic, Popular Technical Reports.
© 2025 PatSnap. All rights reserved.Legal|Privacy policy|Modern Slavery Act Transparency Statement|Sitemap|About US| Contact US: help@patsnap.com