Semi-supervision classification method based on flow shape alignment
A classification method, semi-supervised technology, applied in the field of pattern recognition, which can solve problems such as non-existence
- Summary
- Abstract
- Description
- Claims
- Application Information
AI Technical Summary
Problems solved by technology
Method used
Image
Examples
Embodiment Construction
[0047] In this embodiment, the data set to be classified is called the target domain, and the data set with different but similar category labels is used as the auxiliary domain. The semi-supervised method is used to combine the training data of the auxiliary domain and the training data of the known category of the target domain as new training data, thereby helping to classify the data of the unknown category of the target domain. The present invention will be described in detail below in conjunction with the accompanying drawings.
[0048] Such as figure 1 and 2 As shown, the specific implementation steps of the semi-supervised classification method based on manifold alignment proposed by the present invention are as follows:
[0049] Step 101, based on known category information, establish category coordinates of the training data set in the auxiliary domain and category coordinates of the training data set in the target domain.
[0050] Specifically, based on the categ...
PUM
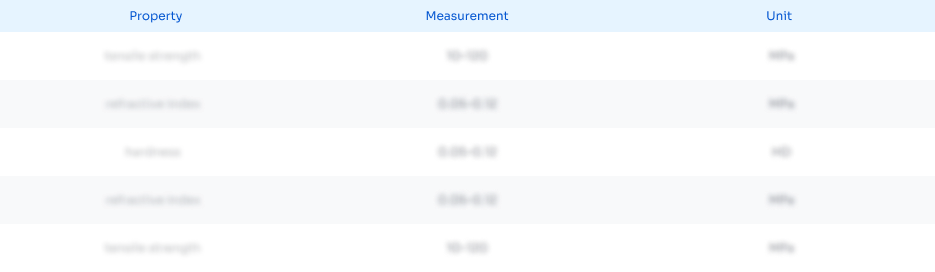
Abstract
Description
Claims
Application Information
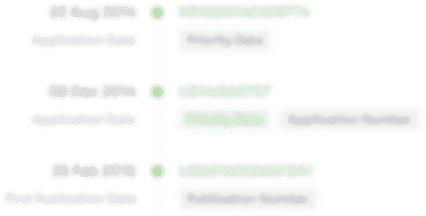
- R&D
- Intellectual Property
- Life Sciences
- Materials
- Tech Scout
- Unparalleled Data Quality
- Higher Quality Content
- 60% Fewer Hallucinations
Browse by: Latest US Patents, China's latest patents, Technical Efficacy Thesaurus, Application Domain, Technology Topic, Popular Technical Reports.
© 2025 PatSnap. All rights reserved.Legal|Privacy policy|Modern Slavery Act Transparency Statement|Sitemap|About US| Contact US: help@patsnap.com