Anomaly Detection Method of Rotating Machinery Based on Dirichlet Mixture Model
A technology of operating state and mixed model, applied in the direction of instrumentation, design optimization/simulation, calculation, etc., can solve problems such as difficult to determine the distribution form, neglect, and reduce the accuracy of anomaly detection, and achieve high accuracy and strong real-time effects
- Summary
- Abstract
- Description
- Claims
- Application Information
AI Technical Summary
Problems solved by technology
Method used
Image
Examples
Embodiment Construction
[0048] The specific anomaly detection process of the present invention will be further described below in conjunction with the accompanying drawings.
[0049] Such as figure 1 Shown, the concrete flow process of the present invention is as follows:
[0050] 1. Separately collect the operating data of the unit under normal working conditions and real-time working conditions: collect data that can characterize the operating status of the unit through sensors installed on the machine. The data includes normal working condition data and real-time working condition data. Each working condition data The number of samples is 50-150 groups;
[0051] 2. Extract data features and construct phase space: extract various features that can reflect changes in unit condition monitoring data, including time domain features and frequency domain features. Using these features to construct the phase space, the results are as follows:
[0052]
[0053] where: x (i,j) (i=1,2,...,n; j=1,2,...,m...
PUM
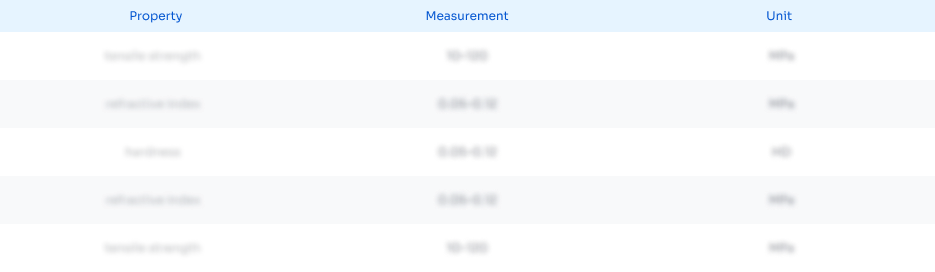
Abstract
Description
Claims
Application Information
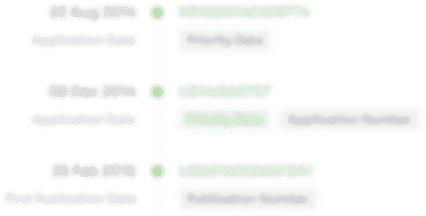
- R&D Engineer
- R&D Manager
- IP Professional
- Industry Leading Data Capabilities
- Powerful AI technology
- Patent DNA Extraction
Browse by: Latest US Patents, China's latest patents, Technical Efficacy Thesaurus, Application Domain, Technology Topic, Popular Technical Reports.
© 2024 PatSnap. All rights reserved.Legal|Privacy policy|Modern Slavery Act Transparency Statement|Sitemap|About US| Contact US: help@patsnap.com