Construction of spectral clustering adjacency matrix based on L3CRSC and application thereof
A technology of adjacency matrix and spectral clustering, applied in the fields of instruments, character and pattern recognition, computer parts, etc., can solve the problems of poor nonlinear data effect, increase the difficulty of fault identification, degradation of corner block structure, etc., and achieve excellent identification effect. Effect
- Summary
- Abstract
- Description
- Claims
- Application Information
AI Technical Summary
Problems solved by technology
Method used
Image
Examples
experiment example
[0081] Data Recognition Effects on Artificial Datasets
[0082] First we construct four artificial datasets, where the data points are distributed on four independent subspaces {Si,i=1:4}, {Ci,i=1:4} are the basis vectors of four of these spaces, where Ci+1=RCi, 1
[0083]LRR and SSC are classic subspace segmentation algorithms; LS3C is an SSC algorithm for constructing hidden spaces with PCA regularization;
[0084] NLS3C uses kernel PCA regularization to construct the SSC of the latent space; LSRC is a robust LRR algorithm; latLRR is the LRR of the kernel PCA regularization to construct the latent space; LRSC is the local constrained LRR algorithm of the PCA regularization to construct the hidden space; proposed algorithm.
[0085] Dep...
PUM
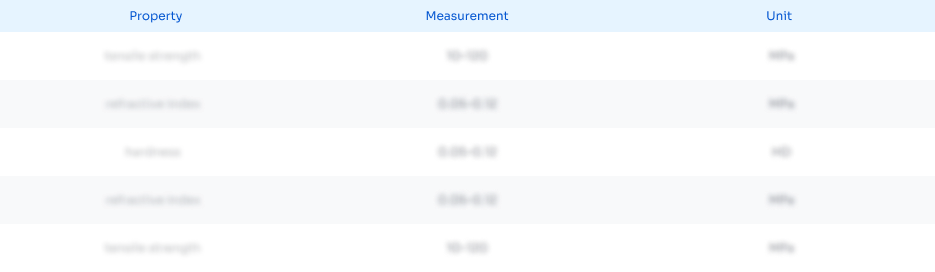
Abstract
Description
Claims
Application Information
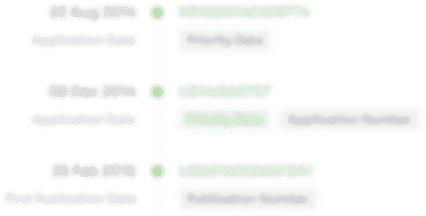
- R&D Engineer
- R&D Manager
- IP Professional
- Industry Leading Data Capabilities
- Powerful AI technology
- Patent DNA Extraction
Browse by: Latest US Patents, China's latest patents, Technical Efficacy Thesaurus, Application Domain, Technology Topic.
© 2024 PatSnap. All rights reserved.Legal|Privacy policy|Modern Slavery Act Transparency Statement|Sitemap