Multi-target image joint segmentation method based on multi-tag multi-sample learning
A multi-instance learning and multi-label technology, applied in the field of image processing, can solve the problems of inability to obtain consistent information, over-segmentation of segmentation results, and low segmentation accuracy, so as to overcome ambiguity and uncertainty, improve segmentation accuracy, and improve segmentation accuracy. The effect of precision
- Summary
- Abstract
- Description
- Claims
- Application Information
AI Technical Summary
Problems solved by technology
Method used
Image
Examples
Embodiment
[0086] In this example, if figure 2 Shown is the input image, through the multi-target image joint segmentation method based on multi-label multi-instance learning described in the present invention, the figure 2 Each image is divided into image 3 The different common objects described in are identified by different colors, and the marks 1 to 3 represent three types of common objects respectively. In the figure, the number 1 represents the girl in the blue dress, the number 2 represents the girl in the red dress, and the number 3 represents the apple and the basket. The specific implementation process is as follows:
[0087] In step 1, object detection is used to obtain candidate object sets of the input image set, and the candidate object sets are clustered to obtain corresponding object labels. As shown in Figure 4 figure 2 In the second image, the result of the object detection of the single image in step 1.1., wherein Figure 4a is the object area obtained by step...
PUM
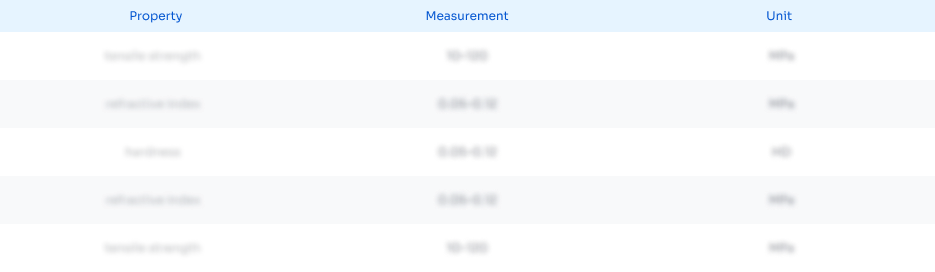
Abstract
Description
Claims
Application Information
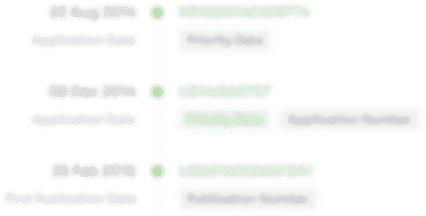
- R&D Engineer
- R&D Manager
- IP Professional
- Industry Leading Data Capabilities
- Powerful AI technology
- Patent DNA Extraction
Browse by: Latest US Patents, China's latest patents, Technical Efficacy Thesaurus, Application Domain, Technology Topic, Popular Technical Reports.
© 2024 PatSnap. All rights reserved.Legal|Privacy policy|Modern Slavery Act Transparency Statement|Sitemap|About US| Contact US: help@patsnap.com