Method for achieving clustering mining by employing parallel weighted affinity propagation big data
A technology of neighbor propagation and cluster mining, which is applied in the field of big data processing, can solve the problems of insufficient data comprehensiveness and insufficient processing time, and achieve the effect of guaranteeing data
- Summary
- Abstract
- Description
- Claims
- Application Information
AI Technical Summary
Problems solved by technology
Method used
Image
Examples
Embodiment 1
[0026] as attached figure 1 As shown, a method for implementing cluster mining using parallelized weighted neighbor propagation big data in this embodiment includes the following steps:
[0027] 1. Build Hadoop cluster platform.
[0028] 2. Divide the large data set into K subsets, and assign the K subsets to data nodes with similar performance in Hadoop.
[0029] 3. Use the AP (Affinity Propagation) algorithm to cluster the subsets. Since the size of the decomposed subsets is relatively small, the central point set Ei={ei, ni} of the class can be quickly obtained, and the Map task is responsible for AP clustering of a subset, and the clustering results are stored in the local disk. Obtain K central point sets for subsequent processing.
[0030] 4. Use the WAP (weighted neighbor propagation clustering) algorithm to perform weighted clustering on these center point sets. At this time, the number of data items in each class, that is, the number ni of points represented by the...
PUM
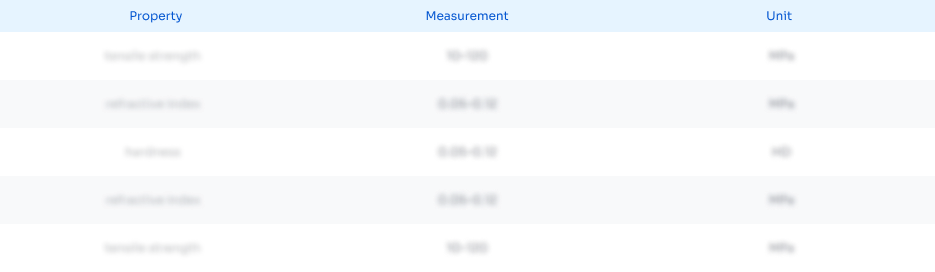
Abstract
Description
Claims
Application Information
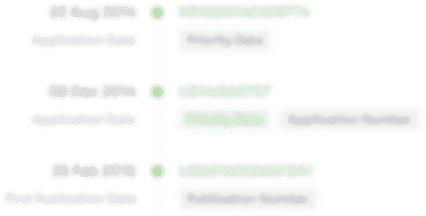
- R&D
- Intellectual Property
- Life Sciences
- Materials
- Tech Scout
- Unparalleled Data Quality
- Higher Quality Content
- 60% Fewer Hallucinations
Browse by: Latest US Patents, China's latest patents, Technical Efficacy Thesaurus, Application Domain, Technology Topic, Popular Technical Reports.
© 2025 PatSnap. All rights reserved.Legal|Privacy policy|Modern Slavery Act Transparency Statement|Sitemap|About US| Contact US: help@patsnap.com