A Robust Recognition Method for One-Dimensional Range Profiles Based on Euler Kernel Principal Component Analysis
A technology of nuclear principal component analysis and recognition methods, applied in character and pattern recognition, instruments, calculations, etc., to achieve the effects of improving recognition speed, improving recognition accuracy, and reducing learning costs
- Summary
- Abstract
- Description
- Claims
- Application Information
AI Technical Summary
Problems solved by technology
Method used
Image
Examples
Embodiment Construction
[0043] specific implementation plan
[0044] The present invention will be further explained below in conjunction with the accompanying drawings.
[0045] The present invention is based on the principal component one-dimensional range image recognition scheme of the Euler kernel to achieve robust recognition in the interference environment. Due to the particularity of the Euler kernel, the original signal features are projected into the complex space of the same dimension without adding too much The amount of calculation is increased, and the purpose of increasing linear separability is achieved. Afterwards, the PCA algorithm is used to extract the main features to further reduce the impact of noise and reduce the feature dimension. It can obtain a higher recognition rate while keeping the dimension of the principal component of the kernel space feature small, which is more suitable for HRRP data than the traditional KPCA algorithm. Real-time processing, the general flow char...
PUM
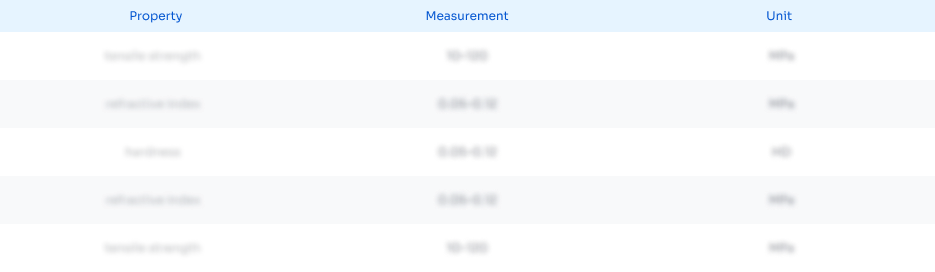
Abstract
Description
Claims
Application Information
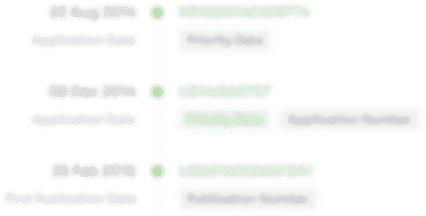
- R&D
- Intellectual Property
- Life Sciences
- Materials
- Tech Scout
- Unparalleled Data Quality
- Higher Quality Content
- 60% Fewer Hallucinations
Browse by: Latest US Patents, China's latest patents, Technical Efficacy Thesaurus, Application Domain, Technology Topic, Popular Technical Reports.
© 2025 PatSnap. All rights reserved.Legal|Privacy policy|Modern Slavery Act Transparency Statement|Sitemap|About US| Contact US: help@patsnap.com