Passive multi-source multi-target tracking method based on dynamic multidimensional allocation
A multi-target tracking and multi-dimensional allocation technology, applied in the field of passive multi-source multi-target tracking, can solve the problems of high algorithm time complexity and low correlation accuracy, reduce time complexity, improve data correlation accuracy, and realize effective tracked effect
- Summary
- Abstract
- Description
- Claims
- Application Information
AI Technical Summary
Problems solved by technology
Method used
Image
Examples
specific Embodiment approach 1
[0024] Specific implementation mode one: as Figure 5 As shown, a passive multi-source multi-target tracking method based on dynamic multi-dimensional allocation includes the following steps:
[0025] Step 1: Using the state of the target at time k-1, establish a preselected wave gate corresponding to the track p of the target
[0026] Step 2: Use the pre-selected gate constructed in Step 1 And the track p at time k-1 and the combination of observation values of each sensor build cost function And construct a binary variable based on the one-to-one correspondence between the track p and the observed value
[0027] Step 3: Use the cost function constructed in step 2 and a binary variable Construct the global association cost function, obtain (S+1)-D distribution formula, and give constraint condition; The number of described S represents sensor, D represents dimension;
[0028] Step 4: Use the Lagrangian relaxation algorithm to relax the constraints of step 3, ...
specific Embodiment approach 2
[0035]Specific embodiment 2: The difference between this embodiment and specific embodiment 1 is that in the step 1, the state of the target at time k-1 is used to establish a pre-selected wave gate corresponding to the track p of the target The specific process is:
[0036]
[0037]
[0038]
[0039]
[0040]
[0041]
[0042]
[0043] in Indicates the estimated value of the target position at time k-1, Indicates the estimated value of the target speed at time k-1, h s (X) is the observation equation of sensor s, is h s (X) the partial derivative with respect to X, is the state transition function at time k, with are the estimated values of the target state and the state covariance matrix at time k-1, respectively, with are the predicted values of the target state and the state covariance matrix at time k, respectively, and the predicted value of the target state at time k can be obtained by predicting the target state at time k-1 ...
specific Embodiment approach 3
[0045] Embodiment 3: The difference between this embodiment and Embodiment 1 or 2 is that the pre-selected wave gate constructed in Step 1 is used in Step 2. And the track p at time k-1 and the combination of observation values of each sensor build cost function And construct a binary variable based on the one-to-one correspondence between the track p and the observed value The specific process is:
[0046]
[0047]
[0048]
[0049]
[0050]
[0051]
[0052]
[0053] where the cost function represents the combination of observations Observations in derived from the objective cost, represents the combination of observations from the target probability, represents the combination of observations The probability of originating from the spurious signal source, represents the empty set, X p is the true value of the target state at time k, is the estimated value of the target state at time k, using the predicted value of the target st...
PUM
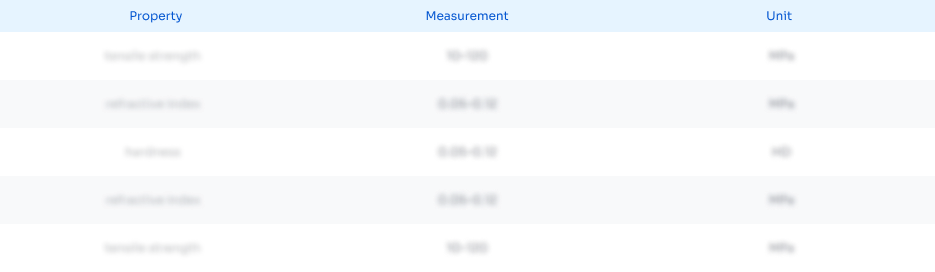
Abstract
Description
Claims
Application Information
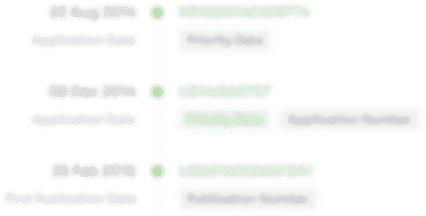
- R&D Engineer
- R&D Manager
- IP Professional
- Industry Leading Data Capabilities
- Powerful AI technology
- Patent DNA Extraction
Browse by: Latest US Patents, China's latest patents, Technical Efficacy Thesaurus, Application Domain, Technology Topic, Popular Technical Reports.
© 2024 PatSnap. All rights reserved.Legal|Privacy policy|Modern Slavery Act Transparency Statement|Sitemap|About US| Contact US: help@patsnap.com