Recording device clustering method based on Gaussian mean super vectors and spectral clustering
A technology of recording equipment and clustering method, applied in speech analysis, speech recognition, special data processing applications, etc., can solve the problems of recording equipment label information loss, lower recognition, equipment damage, etc.
- Summary
- Abstract
- Description
- Claims
- Application Information
AI Technical Summary
Problems solved by technology
Method used
Image
Examples
Embodiment
[0099] figure 1 It is a structural flow chart of the present invention. Including the following steps:
[0100] 1. First, read in the voice sample recorded with the information of the recording device.
[0101] 2. Perform preprocessing on the read-in speech samples, including steps such as pre-emphasis, framing, and windowing of the speech signal. Preprocessing specifically includes the following steps:
[0102] 2.1. Pre-emphasis: set the transfer function of the digital filter to H(z)=1-αz -1 , where α is a coefficient and its value is: 0.9≤α≤1, and the read-in voice is pre-emphasized after passing through the digital filter;
[0103] 2.2, Framing: Set the frame length of the voice frame to 25 milliseconds, the frame shift to 10 milliseconds, and the number of sampling points corresponding to the frame length and frame shift to be N=0.025×f respectively s and S=0.01×f s , where f s For the voice sampling frequency, the read voice is divided into voice frames x t '(n),...
PUM
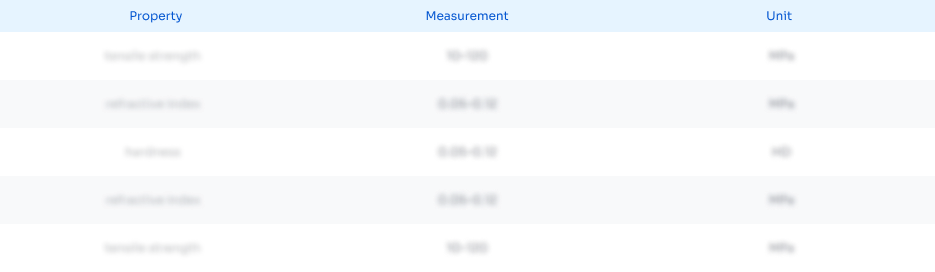
Abstract
Description
Claims
Application Information
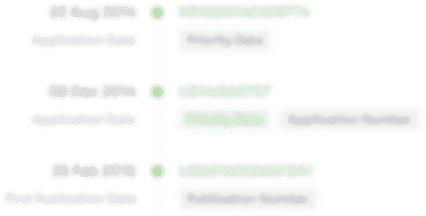
- R&D
- Intellectual Property
- Life Sciences
- Materials
- Tech Scout
- Unparalleled Data Quality
- Higher Quality Content
- 60% Fewer Hallucinations
Browse by: Latest US Patents, China's latest patents, Technical Efficacy Thesaurus, Application Domain, Technology Topic, Popular Technical Reports.
© 2025 PatSnap. All rights reserved.Legal|Privacy policy|Modern Slavery Act Transparency Statement|Sitemap|About US| Contact US: help@patsnap.com